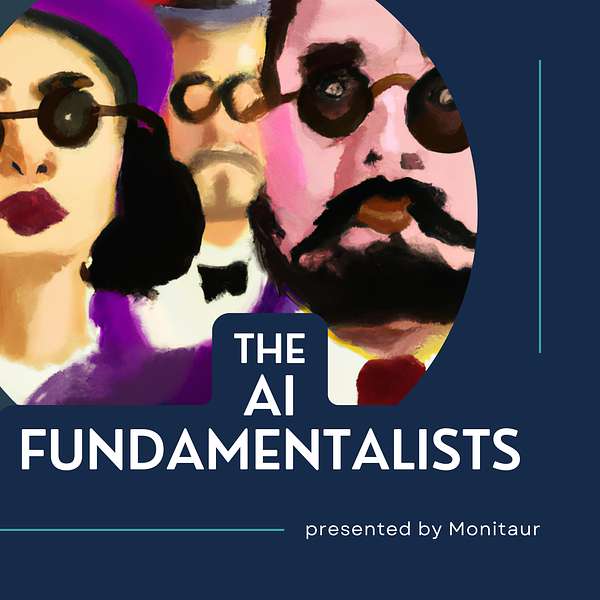
The AI Fundamentalists
A podcast about the fundamentals of safe and resilient modeling systems behind the AI that impacts our lives and our businesses.
The AI Fundamentalists
Why data matters | The right data for the right objective with AI
Episode 3. Get ready because we're bringing stats back! An AI model can only learn from the data it has seen. And business problems can’t be solved without the right data. The Fundamentalists break down the basics of data from collection to regulation to bias to quality in AI.
- Introduction to this episode
- Why data matters.
- How do big tech's LLM models stack up to the proposed EU AI Act?
- How major models such as Open AI and Bard stack up against current regulations.
- Stanford HAI - Do Foundation Model Providers Comply with the Draft EU AI Act?
- Risk management documentation and risk management.
- The EU is adding teeth outside of the banking and financial sectors now.
- Bringing stats back: Why does data matter in all this madness?
- How AI is taking us away from human intelligence.
- Having quality data and bringing stats back!
- The importance of having representative data, sampling data
- What are your business objectives? Don’t just throw data into it.
- Understanding the use case of the data.
- GDPR and EU AI regulations.
- AI field caught off guard by new regulations.
- Expectations for regulatory data.
- What is data governance? How do you validate data?
- Data management, data governance, and data quality.
- Structured data collection for financial companies.
- What else should we learn about our data collection and data processes?
- Example: US Census data collection and data processes.
- The importance of representativeness and being representative of the community in the census.
- Step one, the fine curation of data, the intentional and knowledgeable creation of data that meets the specific business need.
- Step two, fairness through awareness.
- The importance of data curation and data selection in data quality.
- What data quality looks like at a high level.
- Rights to be forgotten.
- The importance of data provenance and data governance in data science.
- Synthetic data and privacy.
- Data governance seems to be 40 % of the path to AI model governance. What else needs to be in place?
- What companies are missing with machine learning.
- The impact that data will have on the future of AI.
- The future of general AI in the future.
What did you think? Let us know.
Good AI Needs Great GovernanceDefine, manage, and automate your AI model governance lifecycle from policy to proof.
Disclaimer: This post contains affiliate links. If you make a purchase, I may receive a commission at no extra cost to you.
Do you have a question or a discussion topic for the AI Fundamentalists? Connect with them to comment on your favorite topics:
- LinkedIn - Episode summaries, shares of cited articles, and more.
- YouTube - Was it something that we said? Good. Share your favorite quotes.
- Visit our page - see past episodes and submit your feedback! It continues to inspire future episodes.
The AI fundamentalists, a podcast about the fundamentals of safe and resilient modeling systems behind the AI that impacts our lives and our businesses. Here are your hosts, Andrew Clark, and Sid Mangala manglik. Hello, everybody. Welcome back to episode three of the AI fundamentalists. I'm Susan Page, and I'm here with Sid Mongolic. And Andrew Clark, once again. And today's topic is going to be about why data matters. Interestingly enough, since our last episode, we got some really good responses about our talk within that episode, which covered MLMs and knowledge graphs. But in there, we had a pretty good discussion about regulation. And since that episode, the EU AI Act has has been passed, essentially, it's been approved for passing people, they the EU really has a solid foundation for what they want to do and how AI is going to be regulated, something for businesses and consumers to build off of. I'm going to take it to Sid to take a look at the regulations because one of the responses that we got was Stanford hai released their report about how foundation models from these big companies will stack up if this act was in place today.
Unknown:What do you Yeah, yeah, so this crfm group over Stanford Research put up this really great infographic, obviously, you know, they did the great work underneath it. But you know, if you want to share and show these ideas to people, we've had to put them in a nice presentation. So we'll link this at the end. But this is a really great graph, which shows you for the major models out there that people are trying to use, like GPT for or palm two, or even the open source llama. How would these models stack up on the current regulations, right, so on requirements on reporting energy consumption requirements on reporting, what type of copyrighted data that you used in your model, and also what type of testing and evaluation you did, and making that open source? And evaluating how these models do on this? So you know, I highly encourage everyone take a quick glance at this if you get a chance. But you know, the the big hitters that we talked about these GPT fours, it scores maybe 50%. Right. So looking at what you want, you know, even these best models are not yet there. What's very interesting to me is so we don't know exactly what the new H uauy aktas, we have the initial draft that came out in 2021, I believe that's, that's very in depth, it's based on risk level and how it's used in very much best practices and model risk management that have been around for a long time. OCC 2011 12, Sr 11. Seven, these things came out of the financial crisis in 2008. And it's the standard that banks are held to and then there's Basel requirements and things other companies, other banks use around the world. This whole like, risk management, Doc data, documentation, model, evaluation, risk management, none of these things are new. The main difference is large tech companies in America and around the world do not do any of these things, because it's a lot of work. And it's the unsexy stuff, the whole purpose of what the AI fundamentalists are about, is doing things the hard way, but doing them properly, where a lot of these limbs and a lot of these models people are talking about these days, they just scrape the internet and grab whatever's there and fed it in. Are they allowed to use this data or how they handle privacy? How do they actually train or validate the whole notion of how a lot of AI is built is very much just like we're gonna go out grab data Swagat and try and push it into something that makes sense. It's very different than like the rigorous statistical modeling that you'll have an actuarial sciences and started in statistics and economics and control theory or or wherever, and evaluation like ML is very much a wild west. So these are trying to kind of rein that in a little bit. So nothing really the EU is doing here is novel, per se, but it's good that they're they're actually adding teeth outside of the banking and financial sectors.
Susan Peich:Now, and I think in the same breath, you know, on regulation, in response to that we saw open API's response and at asking for certain parameters to be removed from the act.
Unknown:I find it a little comical for open AI trying to play in the big leagues a little bit on some of these things, because they don't even have in traditional SAS companies have sought to requirements where it's not a requirement, but it's a voluntary thing that you do that shows you have good internal controls around data management around you know, just general corporate policies, separation of duties, security, and then you know, data retention, all those kind of things, opening, I doesn't do any of that they still don't have a sock to when many companies have those. They also say we're going to keep all your data for 30 days. And they only recently in March said, Hey, we're not going to use it for training. So like just standard practices that other companies already were doing there, and they still aren't wanting to do because they're basically mining your data and building models off of it. So they their their calls for regulation were kind of amusing. When they did that, I thought because it like they don't know what they're getting into. They don't have any idea about they must not have people on the team that know what regulation is, or been in banking or risk management is like you're calling for something you're unleashing a monster we need it. But you don't realize that that's going to destroy your business. They just thought it was like we're big enough, put the guardrails up we can play in here, it's going to prevent competition, but I think it's actually going to backfire on them. Yeah, that's that's spot on. Right. Like, you know, they asked for regulation. And they think that regulation is basically, well, we'll just add some things on our side. And we'll define what regulation looks like. And then that'll just be the world we live in. And when anyone asks us for accountability, you know, we wrote the write the regulations, so we should be all set. And then they are met with people who actually care about data quality and privacy. And then they don't get the outcomes they want. And then they will try and roll it back. very naive and hubris, like where in history have regulations been? Yeah, I'm the big company, I want to write the rules. And I want to make it my way, like, when does that ever happen? I'm just a little curious what what what happened in their boardroom?
Susan Peich:Exactly. And this is exactly why we're grouchy. Let's switch gears just for a little bit. Because the other thread that didn't going out is that cane, also was last week, this week or last week. And, you know, I get a lot of news in my feed about this from a marketing perspective. And, you know, it was really funny to see also in my feed a chief data officer, like kindly shouting out to his peers and colleagues who are going to be going to this event. You know, just remember, AI does three things. When you're listening to these promotions, it finds patterns in data, it makes predictions based on those patterns. And it makes decisions based on those predictions. That's it. I've heard you to talk and I know that that's probably ringing true in your brains, too. You know, Andrew, I think you have some thoughts on this.
Unknown:Oh, I love it. It's very true. It's you go anywhere you go to like LinkedIn is very annoying for me to go to lately, of like, all of these crazy use cases and like how chat GPT is going to change the world. And then like how generative AI is going to just renovate all industries. And it's like, okay, so this thing that's trained off of just random internet chatter that predicts the next word to make it sound like it's a human is somehow solving world hunger. Got it, like we're just in a massive bubble here. It's really crazy. Where that that that post you're sharing is great, like AI does very specific things. I forget his name, who's in charge of of Facebook's AI research group, he's very solid, one of the founders of deep neural networks. He had an article recently on this, like, Hey, guys, like, this is not how this works. He doesn't not like LLM. She's like this has taken us away from human intelligence. So it's very interesting. I'm sure. So do you have some thoughts on that? Yeah, I mean, this brings like the conversation you have, every time you hop in a rideshare app, or every time you're having a conversation at a dinner table, and you have to answer the question. So what do you actually do? And, you know, we would hope that AI was at least understood by AI leaders. But we always see time and again, that that's not what we see. We see, we see AI leaders talking up the big games, we've been getting really excited, we're gonna get really hyped. Talking about old we're gonna replace teachers. We're gonna replace lawyers. And they're always forgetting to fall back on the fundamentals and look at like, what is AI actually doing versus what, what our market is telling you AI is doing. So that this disconnect is always is always very funny. And I'm sure it's a very funny talking point for anyone that's in data science. When they're asked to describe what they do, and why, what you know why these models can't do what you think they can do.
Susan Peich:Yep, for sure. And that's a great segue to our topic for today. And why does data matter in all of this madness?
Unknown:It's great question. And that's why the fundamentalists are here. Right? Well, it's you can very simply, it's, I mean, garbage in garbage out, right. Having quality data we're bringing stats back and stats has kind of gone by the wayside for a while of like, we're all into this, you know, AI algorithm approach to things but really, data is what soak key if you need to have representative data because it doesn't matter what LLM generative AI whatever opening is telling you, whatever somebody else is telling you, data is fundamental for even these types of models are probabilistic predicting the next word for an LLM, based off of the what's the corpus and what your previous words were? Well, how does it know that it knows that from data, it doesn't have a brain, it's not like doesn't have actual learning or knowledge or things, human qualities, it's learned that based off the training data set,
Susan Peich:sit any thoughts on that?
Unknown:Yeah. So you know, let's, you know, going back to fundamentals again, and bringing stats backs, you have to remember how these models work, as we just talked about, it has to find patterns and data, and then replicate those patterns. And so the data that we show it is the patterns that it recognizes. So if we show it, faulty patterns, incomplete patterns, bias patterns, that's all they can give back to you. So the data and the model are one on one, the type of data that you put in will define the type of model that you get out. So these are, you know, fundamentally the same problem. With the caveat that you need to have the right guardrails in place on top of that even because even with the right data, it still won't necessarily do the right things like Susan mentioned earlier about taking away lawyers. Well, there's that big we can link in the show notes a big thing where there's a lawyer in trouble for they did a case filing now, where they're generative AI made up a case that was support their case, and it's completely made up. So they're getting sanctioned, it's a big hole mass will link link about that. But even if they just trained it off of different legal documentation, some of these models that hallucinate it, right, it made up a it looked right, it looked like it would make sense that a case would be this way, but it wasn't correct. It goes back to our Knowledge Graph conversation. Last time, if you were actually have a mission critical no error we need if we are reinforcing a legal case, we need to have actual facts. LLM might not be the greatest idea here. But this is also where like, the whole approach of why we're seeing bringing statspack versus how ml community on AI community often looks at things, it's let's get as much data as humanly possible with what open AI is done. And let's just like toss it in, and the algorithm, we're just going to optimize and it's gonna be great. But sometimes you have bad data in there and understanding what's the good bit data, where's bad correlations? In really understanding what are you trying to get out of this, don't just think of like, Oh, I'm just gonna throw data into it and figure out what I want afterwards. This is kind of the issue and with the EU AI regulation, and things are like, well, what are you actually using this for? How do we know this is proper? It's not biased. It's not skewed, it's doing what you want it to do? Well, you need to understand the use case. And sometimes you need to sample your dataset into something smaller.
Susan Peich:And so with, it sounds like a data data quality to an extent, given the EU AI act, and some existing regulations on data itself, like, is that enough? What what do the data controls that are in place already have to do with this?
Unknown:That's a great, great point. Because a lot of the if people are using using data, which say GDPR, which is the existing regulation, like if you're using data about individuals and things you need to be have them the right to be removed and things like that. Eu AI act is, in my mind, and I'll say comment is a lot less of a new thing. More of like, Hey, by the way, GDPR actually applies here, versus it's been a big fight, there's been a lot of remember when GDPR came out, and I was doing some analysis was in companies is like, well, can we just not do it for for models? If we had training data? Can we just let that slide there's been kind of like a thing people have been talking about for a long time. And they knew they weren't supposed to be doing that. Because like if we have, we can't really remove that. What do we do? Do we have to retrain our models that person opt out? Like, what do we really do here? Versus EUR is explicitly Yes, this person has to consent. And for these high risk use cases, you must really document things. But this is where it's less of the new same with, like, their whole risk management approach is, is from OCC and then the GDPR is really like EU AI is 2.0 Hey, we're enforcing GDPR for your models. Yeah, that's That's exactly right. You know, and in a past life, Andrew and I worked in finance and GDPR was a was a huge problem. Because you know, these are organizations that already have a lot of regulation are already thinking about a lot of problems, and they still have to scramble to get this together. And so it's surprising that the AI field is really caught off guard, that they would be included in this too, that they felt like they would maybe be gonna be exempt from this. But they're going to be subject to the same reviews that everyone else was. And I think that's going to be really interesting to see how that changes. What kind of companies can flourish, what kind of competence are just going to have to fall by the wayside. And it's really, really interesting because that's where you see, like if you go to financial services as an example, they use models in very narrow specifics. They're very, very detailed about what they're doing, why they're doing it, how they're doing it. The validation, the reviews and all that kind of thing is very narrow use cases you come to like silicone rally. It's kind of like, yeah, we're using this thing is doing everything. What is it actually doing? How are we validated? Is that actually work for that we don't know. But we're just going to do it anyway. And that's where it's like, the EUA is kind of like No guys, if it's if you're using this just to predict what you should have for dinner, go for it. And you AI still gonna allow you to do that where you LLM stay or not restricting that if I'm predicting that it has no personal identifiable information, and, and all that I can still use it for that use case, it's for high risk, I am public policing, I'm using biometric information, you can't do it for that kind of thing. So that's where it's also on the surface. It's very obtrusive. But then also, it's not, if you look at like, where are you actually using these things? And where should you be using these things? Yeah, so let's, let's start talking about what those specific pieces look like. Right? So we've talked about at a high level that these regulations are going to be out there. So what are some of the controls that we expect to see on data to meet these types of requirements and the types of controls that other organizations and other fields have been doing for a long time? You know, what kind of regulatory data do we expect? And what kind of reviews do we expect to see on this type of data? Oh, for sure. It's this is data management, you know, is easy, huge thing. There's data governance, there's like a chief data officer, a lot of financial companies, there's whole departments, they have their own software for data lineage, they require certain standards of your data dictionaries. And when you change a data field, and how do you validate that, like, it's a whole thing, Silicon Valley scraped from the internet, were popping it into model. Now that's very different than how Financial Services uses it. Because the existing regulation so like analyzing exactly what inputs you're using, why, what's the provenance? Where did this come from? How do we know we're allowed to use this? Was this from a third party? Does this have PII information? What different laws? So Virginia and California, for example, don't allow you to use this data? But Texas might, you know, like, actually being able to do that analysis and knowing what the data is? Where does it work? How can I use it? And having all of that work and the documentation around it for when someone comes to audit, you improve it out? There's that's what's coming. And that's but for financial companies that's already been here.
Susan Peich:Can I ask a question about data collection? From my point of view? As a marketer? Yeah, a lot of this. A lot of this collection is done by us by Sales by Customer facing people. I've gotta believe that add some dynamic to what company is going to be facing in collection and quality when they're using AI? Can you comment on that? No, I'm,
Unknown:I think the quality will actually go up. It's same with when we had to introduce those cookies, right? So like, almost every website now will say like, do you allow me to use your information and things like that, you have to start explicitly doing it. There's a lot of marketing tools out there, and behavioral marketing and things like that, where it's a little creepy, like the kind of stuff and cookies and like information they're involuntarily getting from people that don't know actually like, okay, they might know, there's some somehow they're being watched, but they don't actually understand what's happening, a lot of those are gonna get reined in a little bit. But the flip side of that is like, so you might lose a little bit of data, but the data you do have will be higher quality and can rely on it more
Susan Peich:interesting said anything to add to that?
Unknown:Yeah. And quality data is not just data that's well collect, it's also well structured, right? And well structured data, looks like keeping track of the types of valid fields that the data can have is, you know, what type of data is it? How often are we collecting it, keeping track of where we got it from? Having some type of check to make sure that it's accurate data. So on top of this massive collection, we also need structured collection, because that structured collection is going to allow you to answer regulators, it's going to allow you to answer questions about the provenance of your data. And it's gonna allow you to effectively audit your own data.
Susan Peich:Interesting. And then is there any example that we can use to illustrate what that does and doesn't look like overall, anything we want to emphasize there?
Unknown:I mean, we could say like one of the bastions of quality, which still has issues as well as like US census. So it's a very structured very like detail. And there's always going to be issues with sampling and measurement error and just the complexity of it, right. But it's a very structured like set of questions we ask everybody, people know what they're getting into, they know what they're supposed to do, sometimes they have to do it, because it's if you're a US citizen, but like, it's a very specific, you know, what it is, you know, how the data is being used, you know, it's being secured. They have set formats for how they, how they put it, how they store it, how like it, that's an example of like a very well done statistical exercise, versus like, Hey, we're just going to get people's browsers history and like, just randomly scraped stuff from their LinkedIn. So we're going to make profiles, like that's, that is going to be a lot less structured a lot of complexities there. And there's going to be some people that don't answer fields and things versus like, the census is very specific, even for a lot of the questions will actually be their category. They're categorical, like, you're in this bracket, this bracket and this bracket or this this, this was like there aren't these weird missing data fields are 28 different ways of spelling the same thing like it's very structured. And so the other type of control that we see coming right off of the census is Basically, representativeness right? The census gives us a sense of what type of demographics do we expect the data to be coming from. And so the onus was on us as data collectors to go back on our data, and evaluate if our data meets the representative goals of the communities that we're collecting from. If we're trying to build models that are applicable to Wisconsin, and we look at our data and our data does not even meet the basic demographics of people that live in Wisconsin, then our model is not going to be representative for that community, it's going to be skewed. And it's going to give outcomes that look more like what we've collected, and not like the actual people that live in that community. It's a great point. And it's a very complex issue of like, you want to make sure because say you go to rural Wisconsin, and it might be very biased towards one ethnicity, or very skewed towards one ethnicity. Well, you still want to make sure that all ethnicities because models are done, right, they only they have to see the right sample, they have to see enough samples to be fair. So you have to sometimes up sample demographics to make it so your model can still be fair, but you still want to be representative that area, because you can't just say you can't just make everything 50 5050 across the board or you don't have predictive quality. And that's also not representative. So it's very complex science. But the good news is that statistics and statisticians have been doing this for a long time, and they have some proven techniques on doing these things.
Susan Peich:represent you have to know your objectives going in. In order to determine test for and monitor for biases, I've got to imagine anything that we can, what else should we learn about that in our data collection and data processes?
Unknown:Well, one quick thing that I'll hand it over to Sid, is you nail it on the head, right there is completely opposite from how open AI and a lot of these algorithms, you're like, you go in with the objective and know what you're trying to do when you curate data for that. paraphrasing what you just said, versus the LLM approach, if let me grab just whatever randomness and just like shoehorn it in and we're just going to put some sprinkle some AI on top of it, it's going to be great. Versus like you have to have that you know, we're bringing sampling a sexy or we're bringing statistics back or whatever we want to say of like, you have to know those objectives. This is the traditional modeling that you'll get where grouchy you get a lot of these talks to statistician actuary economist or grouchy as well like the you need to know what you're doing before you go into it. So that's like the step one for a data bias. Yeah, this and this is really the fine curation of data. This is the intentional and knowledgeable creation of data that meets the specific business need. When we don't do that, we see things like Dan, which maybe a lot of you were on Twitter, with, with chat GPT do anything now, which which really peeled back this like thin veneer of compliance and friendliness to check GPT had, it had been allowed to read everything and had been allowed to, you know, incorporate all this data that it read on, essentially, you know, read it was a large data source there. So it's not these five data sources like Wikipedia, it's also just you know, what people willingly post on the internet with their own human biases, which then these models can then spit back out at us without, you know, this intentional selection of data. We go down one more layer, like it gets very complex in the weeds of like, well, what metric? Are we even looking for? What is fair? How do we how do we what is fair in different contexts, you know, disparate impact is a common metric that people have been using, there's issues with that, because it just looks at the, like, diversity between, you know, applicants and people that that got acts that were accepted, it doesn't, but it doesn't have a notion for where you qualified or not. So there's a lot of all these nuances. There's things like equalize, or just a lot of times, we can do like a full podcast or two on just those aspects. But have using those data sources not dropping it. Another common thing we see in some some industries is fairness, through unawareness, where we're just not going to talk about gender or ethnicity or any protected classes, sex, religion, whatever, we're not going to look at any of that. And we're just going to say we're fair, but because we're not looking at it, well, that's not true either. Because the inner correlations in data. So keeping that data, keeping it well managed, remember lineage, privacy, security, all those things we talked about previously, but then figuring out what's the appropriate metric for your use case, what's actual, what is the fair or equitable in your situation, and your context is where stuff gets really complex and where that backing into the EU AI and, and GDPR is everything we're just talking about here, if you're documenting why you're doing that, and, and referencing established standards or practices, that is exactly what they're looking for. It's not rocket science. It's just a lot of work to to do all these things, but very rarely will you and I'm sure it has happened but very rarely, like Well, someone goes through this whole process and then somebody's gonna come in and say, Nope, you're in violation because I don't agree with that metric you chose for bias. Very rarely with companies putting this much thought and objective review. If you're doing these processes, you kind of end up coming to a pretty good spot. It's a lot it's usually the lack of documentation care validation is where what trips people up more than we did all this work and someone doesn't agree with us.
Susan Peich:salient points because it sounds like you know, objective objectives and what your, what your objective is in the first place is often in the role not just in, you know, in maintaining and managing biases and understanding biases. Your curation and Data Selection is key. And I also want to switch gears a little bit because it sounds like it would also be key in data quality overall. And what's necessary to confirm, let's start talking about that sin.
Unknown:Gen. Let me let me give them this the high level of what data quality looks like. And then you can kind of pick this apart a little bit. And I think that Android both have some stories of, you know, when this doesn't happen. So at least at a high level, here's four examples of what that could look like. Right? So you might not meet data quality, if you find that your data is ultimately missing data, right? For some respondents, you don't get certain data fields, you may have a problem where you collect data, and then you realize you credit you, you know, you mistyped it, you collect it wrong, they report it incorrectly. So there's standard measurement error, you might end up with data that basically represents the same thing over and over, we'll get into why that's specifically a problem. And as we've already talked about a lot, you have to be careful of having imbalanced data. And sometimes that's an intentional process, that you have to go back into your data, look at those protected data fields, and make sure that your data was balanced from the get go, rather than dropping it, saying, well, we never looked at it. So clearly, there's no bias here. But doing the manual process of ensuring data balance, is a piece of this data quality. So these are I think these are the four high level topics I would address in terms of what data quality looks like. Fully agree, that's a great way of summarizing it of those are four key things to look at. And it's kind of the if there, of course, can be other outlier things and things you find. But like, if you get those four correct along with the I understand my use case, what I'm doing and document how I got there, you're going to be in a really good spot.
Susan Peich:Now and talk to me a little bit about there's a couple things you said earlier, and some things now. I'm missing data, historical data. And like we talked about earlier, the right to be let go. Talk to us and talk to us in terms of that in relation to data quality.
Unknown:Yeah, the rights to be forgotten is that you know, one of the hallmarks of GDPR, which will be inherited in the EU AI, that's what makes this a little bit more complicated. And what specifically a big pain for, for AI type companies is because if this person has given you like they did the US Census, let's just keep rolling with that you can opt out of the census. But for sake of argument, you can't, right. So you put your information for the 2020 census, and you decide you call up the US government and say, Hey, remove me right, and remove my data point. So anything that ever is connected to the US Census has to go and delete that data point. Yes, probably not going to happen. And of course, government data will never have to have that. But let's just say for the sake of for argument. So you have to have that really robust like that data provenance, that lineage, that data governance aspects to know exactly where everything is, you know, have a data warehouse, where you're pulling all your data from, when somebody opts out, you basically have to retrain your data after you've removed that dataset, pull it in, make sure your data, like you're not skewed, now you don't have anything else. And then you retrain your model. So this is where that huge GDPR was a real pain for companies to implement. But if you're just deleting somebody from a database, that's not that bad. It's that whole retraining aspect and verify and then re verifying even your data quality, and before even retraining. But then training, you know, takes a long time for some of these models. And you know, where that goes wrong is you have modeling techniques, which don't account for this. And so you have this really great model, and you have your salespeople go and talk to clients, and they collect data. And oh, I forgot the age. Oh, I forgot to collect their address, oh, I forgot to collect their reported gender. And then you pass it to the modeling team. They say, well, we can't work with this. You've you've actually just missed data. And like, you know, I've seen situations where I get a data set, and I'm told to model it, and over 50% of the data is missing at least like two or three columns. And you just can't you just can't work with that data. And that's, that's horrible, right? You spent all that time and effort. And without having that emphasis on getting all the data having complete data, you have to throw away a lot of data. Fully agree. There is a kind of a segue here for another podcast potentially where we can do a full podcast on synthetic data. There are some cases where do you know for the privacy concerns and or if you're missing too many columns where you can interpolate or extrapolate or like infer certain columns in for certain data sets or You have a really protected data set, you can actually just model the distributions of individuals on top of it. And then you model off of that you get the same relationships, but you're not actually using the individual. So like, there's a lot of things you can do. And it's not the end of the world that open AI might be saying, you know, if you get this whole representative dataset on individuals from Wisconsin, well, we can actually model the distributions of the total outcomes. And then we that's not protected. And if if, if I removed myself from that Wisconsin dataset, well doesn't change the distribution, you can still use that data sets, there are actually workarounds to it's not this apocalyptic end of AI either. It's there are other techniques, where it's just like this is where things are very complex, and you need to then if you ever do these synthetic techniques, you need to be very, very, very cognizant of what you're doing. And then truing back, okay, I made this synthetic data set, then we do really need to get that census data and confirm that the properties of you know, imbalance or bias and all those things that we want to ensure that are representative still are.
Susan Peich:Let's say all things that are perfect. In all things are same in the data collection and the governance of the data and what you're using to train the models. What is the path that like, that seems to get us 40% of the way there on an LLM or any type of machine learning model? What else needs to be in place? Very practically speaking, like, what are companies missing? Because there's a party line out there? I'm going to paraphrase it that? Well, if we use quality data and fence it at the data level, and that's good. That's half of our governance, the concept came up in the context of somebody talking about model cards. Yeah,
Unknown:that's a great point. Well, if you think about like a model lifecycle, you know, you have business, understanding data, understanding data prep, and then modeling, evaluation and deployment. So a lot of that chunk is you got to get really good data and understand your use cases, and then you're just modeling with really good guardrails and evaluation on the back end. So that is definitely key. The part where it gets interesting with like data cards and model cards, is Silicon Valley's they really came out of Google, they Silicon Valley's attempt of we're doing governance, we're making a baseball card and our whole LLM model can be summarized in two paragraphs of bullet points. And that's all you need to know. And you can know where responsible and accountable. Now, I'm not saying that's exactly what Google is trying to say. But when the concept of, of when, like a model card comes out, and that's sufficient governance, as we've talked about, what what entails every step you need to go through, I can't fit that on a little tiny sheet and say, Oh, this is all I need to do. It's a long document that looks OCC type. So model cards, and debit cards are definitely a step in the right direction from when SIL when they came out, you know, five years ago, or so when they did when Silicon Valley was doing nothing. It's like, Hey, we're doing something. But it's more of an FYI, for developers, I would say versus the governance, we have covered a lot of the key aspects of governance and the data part is very huge. But the amount that we've talked about it like you need to document that is different than the data card, like the data card model card does not solve that. And if a company is like I'm going to solve it with model cards, well, now that's a nice TLDR readme. But that's not the same thing.
Susan Peich:Great, the greatest editor now is TLDR on model governance. said anything to add to that.
Unknown:Yeah, I think that there's like a lot of, there's gonna be a lot of pushback to GDPR. And you know, you can almost see model cards as a precursor to this, which is like, just let me do what I want to do. And let me just do the bare minimum. And there's going to be a heavy interest of just trust me. And mandala cards are a great example of just trust me, which is like, well, you don't have to know how the sausage is made. It's fine. It's already been served for breakfast.
Susan Peich:There's just so much more to explore. And I want to make sure that our listeners they're taking away, just really some key points about, you know, the impact of data is going to have which I think you guys have summarized very well. And you know, what can you do? What can you do now? Andrew, I think you have some thoughts on this? Yeah, well, I think we can actually
Unknown:in this on a happier note, because despite what open AI or anybody might be saying, we've already talked about you can use synthetic data, you can do these processes, the fact that company is going to have to take a step back and sit and there's been calls by different people about like the suspension of general AI until we get our stuff together because it's apocalyptic or like I don't believe any of that stuff. But it is true of like if companies take a step back, and actually what am I building? Why am I building it? Do I have representative data and build it off of those processes and talk to any data scientist that knows what they're talking about? You can use synthetic data and things. It's not the end of the world. It's not like AI is stopping now. I would argue that companies are going to whine and complain about doing these things. It's going to prevent some of the big tech companies from having as much of a moat as they have now, but it's going to allow smaller companies with domain expertise we're going to actually see in five years a lot more use For generative AI solutions, and we're seeing now, open AI might not be this, like, chat GPT is not going to be a thing in five years like these models move so fast. Like where there might be new chat ups, it's not going to be the version we have now, opening is not going to be the only one. We're already not the only one. But we're going to have actually more useful solutions because people had to take a step back and say, Why am I doing this? How do I know my data is right? How do I know it's not biased? Like all these things people are like really concerned about? This solution is very simple, but it's very tough. What the fundamentals are about it's not fun. Go read OCC, it's it's a solved problem. That's what he was gonna make you do. But it's going to make your models better. It's going to make them more accurate. It's going to make a better experience for consumers. Yeah, and I think that's exactly right. Like, you know, we there is room for optimism. We've talked about a lot of problems in this episode. But these are all resolvable problems. These are all problems that can be addressed and anticipated and work through with the correct process with mindfulness with the correct documentation. And you know, Andrew, and I wouldn't work with AI for so long. If we hated it. We really like AI. And that's why we're here. But we want to see people do it right. And doing it right means doing it the hard way.
Susan Peich:Excellent points, both of you for our listeners. Thank you for joining us today. If you have any questions for the fundamentalist please let us know. Otherwise, this is Susan Syd and Andrew signing off for today.