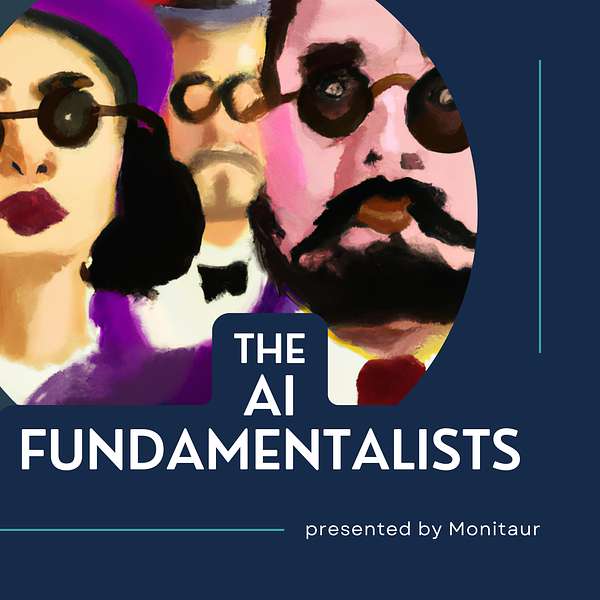
The AI Fundamentalists
A podcast about the fundamentals of safe and resilient modeling systems behind the AI that impacts our lives and our businesses.
The AI Fundamentalists
Upskilling for AI: Roles, organizations, and new mindsets
Data scientists, researchers, engineers, marketers, and risk leaders find themselves at a crossroads to expand their skills or risk obsolescence. The hosts discuss how a growth mindset and "the fundamentals" of AI can help.
Our episode shines a light on this vital shift, equipping listeners with strategies to elevate their skills and integrate multidisciplinary knowledge. We share stories from the trenches on how each role affects robust AI solutions that adhere to ethical standards, and how embracing a T-shaped model of expertise can empower data scientists to lead the charge in industry-specific innovations.
Zooming out to the executive suite, we dissect the complex dance of aligning AI innovation with core business strategies. Business leaders take note as we debunk the myth of AI as a panacea and advocate for a measured, customer-centric approach to technology adoption. We emphasize the decisive role executives play in steering their companies through the AI terrain, ensuring that every technological choice propels the business forward, overcoming the ephemeral allure of AI trends.
Suggested courses, public offerings:
- Undergrad level Stanford course (Coursera): Machine Learning Specialization
- Graduate-level MIT Open Courseware: Machine Learning
We hope you enjoy this candid conversation that could reshape your outlook on the future of AI and the roles and responsibilities that support it.
Resources mentioned in this episode
- LinkedIn's jobs on the rise 2024
- 3 questions to separate AI from marketing hype
- Disruption or distortion? The impact of AI on future operating models
- The Obstacle is the Way by Ryan Holiday
What did you think? Let us know.
Do you have a question or a discussion topic for the AI Fundamentalists? Connect with them to comment on your favorite topics:
- LinkedIn - Episode summaries, shares of cited articles, and more.
- YouTube - Was it something that we said? Good. Share your favorite quotes.
- Visit our page - see past episodes and submit your feedback! It continues to inspire future episodes.
The AI fundamentalists a podcast about the fundamentals of safe and resilient modeling systems behind the AI that impacts our lives and our businesses. Here are your hosts, andrew Clark and Sid Mungalik. Hello everybody, welcome to today's episode of the AI fundamentalists, where today we are switching gears a bit from talking about modeling best practices themselves to getting into a discussion about upskilling the people behind the modeling best practices that we're putting into place every day. And just today, as the day of this recording, linkedin released their top 25 jobs on the rise for 2024. And it's just a reason. The positions in the top 10 government program analyst was at number two. Sustainability analyst was at number five, and I think this is significant because it's putting data and data analysis in the line of business or into practice. And then, rounding out the top 10, at eight and 10, respectively, were artificial intelligence consultant and artificial intelligence engineer.
Speaker 3:I mean I don't fully agree with a lot of these lists. Anyway, I definitely I see the artificial intelligence engineer. What do they really mean by that? Do they mean prompt engineer or do they mean actual engineer? So, like, these are kind of squishy titles, I think, but I think the thrust that you're getting at is that how can companies better leverage AI, and the focus on that will 100% be something in 2024. I'm not quite sure personally what an AI engineer is, because that's very, very broad, but this is a major focus for companies, I definitely think, is what that entails, like machine learning engineers, like we would do the ML ops and stuff around that, and then the prompt engineering and software engineers that can use API and stuff. I think that's definitely what they're kind of saying and I fully agree.
Speaker 2:Yeah, this feels like a follow up of like you know, it was like six years ago. We got like the hottest job in the market is data scientist. This feels like just like the newest version of that and this AI, consultant, engineer world like these are not like roles I've ever seen. It really is the ML engineer, it's the data scientist, it's the software engineer, comma, machine learning, or it's a research scientist. So this kind of feels like a catch all umbrella for you know the remnants of the data science craze which is still here universes of adding data science programs, like very recently. So I can see the push being there, but it's a little too abstract.
Speaker 1:Yeah, I was actually. I mean, remember this list is all jobs, all industries, according to LinkedIn and their recruiting day and their hiring and recruiting data. Also of note in that list were recruiter, director of legal operations and workforce development coordinator, which doves tails nicely into some of the other things that are happening in the you know, to support we're going to talk about later with upskilling, and that's just the organizational disruption that's bound to happen, as people have figured now that they've had a chance to play with AI and see some of the things that it can do. Now there's going to be this realization that so where do we use this, what are the use cases, where are we going to apply this and what skills and therefore mindset needs to be in place in order to, you know, see actual results versus the perceived or marketed results that we're told about.
Speaker 3:I think that's great and that's a very good segue as well. From what we have here is, I hear the thrust of what this list is saying, but they're getting it all wrong. And this is kind of what we had in the data science craze as well, as people hire a ton of data scientists, which were basically glorified data analysts. You hire a bunch of them and you here's some data, go have fun, but then there's no way to actually action that. So I think what it more appropriately which we'll get into for today's podcast is this specific disciplines that you need to have experts in. And then there's a couple expert generalists not too many of them, but a couple that know how to kind of connect the dots Less than these nebulous AI engineer. What is that, I don't know, but we do know what an operation research person is. We do know what an econometrist is, what a statistician does those specialties with somebody that can kind of guide the principles of what's possible and where to apply. That, I think, is definitely key.
Speaker 1:Exactly and really, and when we think about bringing those all together as an organization, we also have to remember that, as we're going to talk about in this episode, it's also helpful to understand that, no matter what the role is, even the ones that are making AI today no one is going to be immune from learning new things about your craft, new skills that force your craft and, overall, new ways of doing your job, and that is, and always has been, table stakes to being successful in your role and growing your career. Andrew, I'll start with you. Do you remember something as significant as this or something in your career that really put you in a vulnerable place to either shift your mindset, shift your skill set quickly from a lot of the things that you knew?
Speaker 3:I haven't really had too much of that situation as I've kind of basically my whole career been pushing on the technology on that side of the house. But I know one thing when I was coming into the workforce was really the transition to moving everything more to cloud, and still companies are still working on that now, really the oh my cloud's a thing or dropbox is a thing. How do you prevent data loss and all those kind of security considerations? I started working as an IT auditor initially, so really there's a proliferation of cloud sources and then trying to get your own customer data on there and there's a huge disruptive pattern for companies. I think there's a lot of great information that we can learn from that era as well, as we're looking forward. Here's sort of like the shared responsibility model of who manages what as you move into the cloud and all those different controls you'd have in place, and I think there's a lot of good parallels here with the AI revolution.
Speaker 1:No, and Sid, how about you?
Speaker 2:Yeah, I mean, when I started my journey as an NLP researcher, we were like linguistics, people, right, we were computationally like, we knew about parsing, we knew about sentence structure. We were thinking about, like, how does a sentence form by a human being? And with the not advent of transformers, but the pick up of transformers, which has developed into this new world of generative AI that we see everywhere, I had to totally change from someone that was a computational linguist to a deep learning scientist. The world, you know, the research world, has been fast and ever. Now. I think the last five, six years have been more productive than maybe the 10, 15 before it, and so you always have to be on top of what your field is doing, otherwise you're going to get left behind. You know you can't really do with LSTMs that we do now with transformers. Yeah.
Speaker 1:And you know, similar to Andrew I mean I was. Probably the most significant thing I remember is my first job out of undergrad in the late 90s. That was also the dot com boom. So yeah, I had to remember like I had one skill set come out of college, that was occupational therapy. And then here was the. This is just a mark of how fast the technology was happening.
Speaker 1:At that time we had a manufacturing, a steel manufacturing company, coming to our university and saying we don't care what your major is, here's this logic test. Take it, you pass it, we will teach you COBOL to help us get off of all these applications, off of the mainframe green screens and into computer software. That was even pre-cloud, I mean, this was called client server software. So not only did I just date myself with that, but you have to understand that, like, big disruption has always been happening and even when it seems really obscure, there's going to be a mindset shift because the environment is changing and you still bring something to the table if you're given a chance to take a look at it in a different way.
Speaker 2:Yeah, so let's let's hop into upscaling here. So we're going to talk about upscaling from the perspective of you're already in this field or you're just outside this field and you want to learn more about AI fundamentals. You want to start building models thoughtfully, with safety, fairness and robustness in mind, and what can you do and what can you learn and what can you bring to your organization to really show that you're going to be part of this revolution where we're going to make fair AI, auditable AI, explainable AI systems and get them out there into the world? So this is going to be the types of skills you need to adopt and think about to do this type of work, which maybe you don't already have. So we have a bit of a hierarchy here and I'll let Andrew get started here, but we have a couple of positions and how they can approach this.
Speaker 3:Yes. So for today, we're going to talk about basically from being the closest to being an AI fundamentals practitioner to the furthest we're going to talk through data scientists academic, marketer, auditor and then executive and we'll kind of level of detail will vary between groups. So we kind of want to give that spectrum as you kind of get farther away. And what are some key areas to focus on and potential areas that, based off of the personas where we're familiar with you might want to try and expand your knowledge. And, of course, this is a generalized guide, does not apply to everybody, but hopefully some good ideas for different areas. So, for, as a data scientist, one of the key things that the scientists often have is a lot of them came from different fields and can you kind of slide into this this data role, going from analysts learning how to program that kind of thing? A lot of data scientists have really started with that machine learning style paradigm where that's the one tool in your toolbox and everything's machine learning.
Speaker 3:The problem with machine learning as it's practiced by a lot of data scientists is like I don't understand my data, so I'm going to use machine learning, it's going to make it model. It's going to be great and kind of approaching it that way, versus having any sort of an academic grounding in the different types of modeling paradigms. That are tools in your toolbox, such as, you know, bayesian modeling, frequentist modeling, different types of statistical like those are different types of statistical modeling, but econometrics or control theory or different sub domains where you normally come up very codified way of thinking of the world. There's good and bad things there. People in those domains need to look at other areas. But data science has really come about on, like the need for people to analyze data and make models. But the problem is in pushing to that direction you've missed a lot of the fundamentals of understanding how the different disciplines work.
Speaker 2:Yeah, that's great, and so that really boils. That also boils down to thinking about becoming multidisciplinary, right, we are more than just machine learning people. We are data scientists, and that means that we need to be on top of statistics, optimization. And now in this, in this landscape, trying to make fairy I systems, we want to be thinking about systems engineering and also software engineering. Right, maybe for too long we've been a little bit separate from the engineering teams and we don't really know how these models get put into the real world, how they, what they, how they interact with data, and so we want to get a little bit closer to that world, so the models that we build live in that world more naturally, right, so that might mean you know, learning CICD, pipelines, learning Docker, learning how to you know, make the model and get in front of people, and seeing what that pipeline looks like, so that you understand what's going to happen to your model after you develop it.
Speaker 3:That's a great point and that's really how I'm seeing a lot of the general term data science industry kind of coalesces on. We want a one man band that can kind of one woman band that can kind of do everything, basically make a model, present it, deploy it. That's not a really good, that can kind of work for like alpha MVP type stuff, but long term and that's good. There is a definite need for that expert generalists that can kind of know how to concoct the whole solution. Also, if you are a very deep Bayesian modeler, data scientist, you should also learn those other areas. There's really that two approach, that that generalists that knows enough to be dangerous in those different areas. And of course you want to be a know, learn the different disciplines and learn programming, learn the modeling. And then there's the be very good in one specific area and then know okay, I'm going to have to work with engineers, how do I talk to language, how do I start moving something in Docker? So it's really those two paradigms off from the data science world that we see.
Speaker 3:And depending on what your background is, if you're an academic that's really strong in one area, you probably want to focus more on the programming skills. If you are a programmer, analyst the person you probably want to focus more on learning, specific modeling things. So data science as a term, is that really that generalist, and then that's. I think Sid might have some ways to close up on this. Then we can start talking about some of the research scientists, which is a little bit different. But as we're kind of seeing in the industry, data science as a term is kind of that generalist. That's not necessarily an expert in that many things, but it could be helpful for them to prototype and then hand off to research scientists and engineers.
Speaker 2:Yeah. So I'll just close out our data scientist section with a few specific things you should do. If you're not already in cloud, learn to use cloud. If I had to recommend one, it's going to be AWS. Most of the modern internet is built on it, and whatever you learn there will be transferable to other clouds you work with. If you only know R, it's time to learn Python, and if you only know Python, it's time to learn R and maybe even Julia. Right, learn about how these models work. If you know Python, you have your models, but R has much, much richer history from being from statistical modelers, and those are some great libraries to be able to run. And before we happen to research, research scientists, data scientists should learn to read papers, right. You shouldn't just use tools, you shouldn't just read documentation. You should understand how your tools work. You should understand what they do and how they work, because it's still a very new field. So we want to make sure you understand what we're doing and not just be running code.
Speaker 1:One question before we move on to the research scientist. In specifics for data science, how important is it to also have domain expertise in the data that you're studying? Is it better to be objective or to have some expertise?
Speaker 3:I think definitely, so you can be objective and have expertise, and that's, of course, the golden thing.
Speaker 3:So you really need to be an expert in the field, and that's one of the issues that we've had is, often you parachute, drop data scientists in that don't necessarily understand the industry, and there's so much context in data and industry knowledge that I really think there's a big opportunity for you to be an expert in oil or gas or an expert in manufacturing or something and really becoming a data scientist there, versus data scientists like to flutter around between industries and stuff but then you're never really going deep.
Speaker 3:So I think it's a great point of like there's being deep in stats, being deep in these different disciplines, but also being deep in domains, and those are very important. So there's a lot of areas. As a data science, you have a wide swath, but choosing a couple is very crucial. As our friend of the program, christoph Mollner, said in his book, is be a T shaped modeler. I think that's a great idea. Is have a wide. You know you have a solid surface area, but you're very deep in a couple areas and I think that's really the best way to be a data scientist, and domain expertise is 100% one of those key pillars.
Speaker 2:Yeah, and the practical level of that is if you, you know, say you work in the finance industry, talk to some underwriters, really get to know their world, really understand how they operate. If you work in healthcare, try and talk to physicians if possible. You're not going to become a doctor over the course of a project, but at least you'll understand a little bit of the actual problem rather than just assuming that the numbers work how you think they work. So domain expertise can be achieved from experts, just talking to the experts in your organization.
Speaker 1:Excellent there. Now we can move on to the research scientist.
Speaker 2:So for the research scientist, this is someone who has presumably completed a PhD program, is now in industry, is now working with these types of problems. You probably feel like you have a pretty good handle on machine learning. You probably even have some domain expertise. What's left for you to learn? Probably the best thing for you to learn is to get back into systems engineering. A lot of your PhD background has not necessarily prepped you for the engineering world and what it means to make robust and strong and consistent models that can exist for months or years. So this is going to be going a little bit back to some of the early episodes we did about the NASA researchers and thinking about how you can build models that are built to last and models that work how we expect them to work. So, moving a little bit back towards the engineering side of things and even further from that, say, you're an academic where you've done the PhD and you didn't go into industry. You continue to work in academia as a professor or as a researcher A big disconnect.
Speaker 2:I see there is a lot of misunderstanding about real world use cases and this is where researchers, while they do amazing work, can get a bad name because they do work, which feels disconnected from the problems that people are working on. If you build a system that's for 40 years in the future, no one will use your research. One day they will use your research and they'll be happy that you published, but if you want to upskill and start making impact now, it's time to talk to people that are practicing, people that are in industry and understand what are the challenges they are ready to face today and helping them make solutions for those problems. And that probably looks like learning specific software development skills right, Going beyond research, learning how to build the models yourself and then exploring the pain points that people are expressing and addressing those pain points if your goal is to contribute today to this work.
Speaker 1:Interesting. So it looks like the academic and research scientists are they probably more in tune to having come from a certain discipline or area of expertise?
Speaker 2:Yeah, so often what happens with research scientists is that they'll have done their PhD in biomechanics or in statistics or in math and then they want to go out and work in industry, so they'll work in a more general version of what they learned. If they were doing like routine modeling, they'll still be doing modeling in industry, but then they would be a good fit in the healthcare environment. That's usually where they end up Working under the more umbrella version of their work, computationally, with some expertise that they gained during their academic time.
Speaker 1:Anything else we want to solidify before the academics, the researchers, the data scientists, before we move in there.
Speaker 3:For all of those individuals. They can get siloed very easily. Really, what we're trying to say is figure out where you're wanting to go. If you're an academic or research scientist, definitely the systems engineering, understanding the broader context, understanding the industry, is key, kind of get out of your silo. As a data scientist, sometimes it might be getting a little deeper into an area because you're too broad and you're not an expert in anything. So it's really understanding the context, because context matters Specifically, like you called out, domain matters. So that's really integral across anything.
Speaker 3:If you want to have something as academic, if you want to have something applied to the real world, you can't just do with theory. You have to apply it to a use case. Most people work best and understand the use case in their area. So I think that domain knowledge and understanding the context and use case is crucial for everything. We see so many AI products that are just agnostic across anything, but that means they're not very useful. So having domain specificity, having this mission today, is definitely the best use case that is applicable across all of those different disciplines.
Speaker 1:Shifting gears a bit into the marketer and I have to tell you guys, this one was hard to narrow down because in the steps of the roles as they move away from the core AI building, marketers are marketing AI or products built on AI, but marketers are also proportionately the biggest consumers and buyers of AI for a business Third party AI, in particular, to help understand customer data, help understand how to reach the market. So, breaking this down at the top of my thoughts, if you're keeping an AI buzzword bingo card, add trough of disillusionment to that bingo card. That's a statement of the market and it's personal for me because this happened twice last week once in my marketing cohort as we were being presented some of the predictions for what to understand about the market and their behaviors for this year. Then, all the way over to the other side, the general business of insure tech, it came up again how are you marketing AI products to into that field and to insurers? When you hear it twice, we know this is going to catch on, but really it is the downswing after the huge hype of 2023, and then watching it level out somewhere in the middle. Where 2023 was, the year to play, 2024 is.
Speaker 1:In my opinion, this is going to be the year to prove, as we create AI and see how our customers are adopting it, and or as we're buying AI and we're figuring out. Yes, this is a place we want to automate. No, this is nice to have, but it's not a place that we feel comfortable delegating fully to a machine right now. I think that's one of the challenges for marketing and really understanding the customer. That's going to be one of the biggest challenges. I do want to kick it back to data science and the model builders and the AI product builders. In those roles, what's been the biggest challenge that you guys think with marketing hype, that challenges the roles that we had closer into the stack the data science as the researcher and the scientists.
Speaker 3:That's a great point A little bit of a boom and bust, I think and that we keep having these troughs of disillusionment and things. The marketing hype is taken on a life of its own where you've now overblown what the technology is capable of. Then, when the modest gains of the technology is capable of underdeliver from expectations, all funding is cut and things like that. It's hyped up into something. It's not such as this whole LLM thing. They're predicting the next word. It's not thinking there's nothing fancy going on here. It is helpful for drafting emails and doing things like that with a human in the loop, but it's not automating key business processes.
Speaker 3:When you get marketers and not only marketers but everybody that's starting to blow this out of proportion. It ends up hurting the data science teams and might be given a huge budget. Then they all get laid off when they didn't do what they were supposed to do. But also the challenge of how do you adequately message what the technology is capable of? That's something I'm personally trying to figure out because a lot of times people executives I love to say the Gartner tax Gartner as an example. They say something and then it gets in executives ears that that's reality when it has nothing to do with reality, then nothing that. How does the data scientist or research scientist actually say, hey, I'm the expert that actually wrote the original paper. You guys are saying something else, but now you're telling me I'm wrong and I wrote the original paper. How do you combat that? That's an open, outstanding issue. That, I think, is the hitting industry in general.
Speaker 1:There is a good article and we'll put it in the show notes about the three questions to separate AI from marketing hype, and it really is targeted to what to ask whenever you're buying AI and it really is the three pillars of when you have a use case. Go in with a strong use case on what you want to solve for your business and why you think AI, why you think a model-based system can help solve it, and then really start asking a lot of questions how does the model learn and improve? Unclear answers to this question right away from the vendor. It should be a red flag for you because it's either how much of it is truly a model based product, and knowing that could lead you to try and make sure that you try and look at some other solutions that might be present less risk and be less of a black box into the problem that you're actually trying to solve. Some other questions to make sure you're asking is you know, want and know for your business how is the AI managed, monitored, adjusted? The other thing that you'll want to know is like you know, do you share customer data to train the AI?
Speaker 1:And this one is a good one to ask because sometimes you might be buying a product where you do want some introduction of third-party data to neutralize what your own biases and customer data are saying, and that might be the use case that you want to improve. You might also be looking at that product because you have highly sensitive data that cannot mix it all. So don't just say like, if the training on customer data, that's bad, you want to know the goals you're after so you get the right thing. My take is just that there's a lot of pressure on businesses to just adopt AI for AI's sake, but at the end of the day and I think we'll see this year that you know budgets are finite and perceived value is not the same as actual value and businesses are going to be, and when you marry that loop back to budgets are finite, you're really going to want to understand. You know it's real, so value you don't know that use case that you want to solve before you go in and start buying model-based systems to try and solve them.
Speaker 3:Oh, I think, I think, fully agree with that. I think the party, the music, is about ready to stop and there's definitely not enough chairs for everybody when it stops. There's definitely. So I often get heat for like. I think that you know I'm always kind of pessimistic on AI. That's not true. There are 100% use cases for it. I don't agree with the current buzz around it. There are definitely use cases with it and going in with that exactly why do I need this and how does this actually solve something? What can I do today? Versus just buying the hype and feeling like you're going to be left out. That's the problem. Versus like I actually see a specific use case for this. This is why I'm purchasing. It Completely makes sense.
Speaker 1:Now that we've kind of solidified who's buying the AI and who might be bringing in more models, that's a good segue into the auditor role and some of the compliance rules.
Speaker 3:Definitely, and this is we've seen this before, or I see this a lot anyway, because I'm, you know, a former auditor and I still kind of interact with the industry. Auditors are often, they kind of feel left out as well, and auditors want to have a piece of the puzzle and oftentimes they want to, you know, be in the action and things like that. And one of the key there's two carriers I'm constantly talking to them about, which is number one you can't automate audit. The whole purpose at first line can be it should be, you know, exploring these automations. And how can we start making our jobs more automated, more efficient, right? Second line, which is the ones that define the controls there you can maybe make your process more efficient at times. But the whole purpose of the third line of defense is basically like your safety and football or something. You're the person to make sure that that running back, running back, there we go, the running back doesn't break through the defensive line and then and make a touchdown. The safety is there to try and prevent that from happening, right? That's what internal audit is supposed to be. They're supposed to be the ones that like, hey, let me take that 5,000 foot view or 50,000 foot view and let me look at and survey the scene and think analytically and critically where could be a potential issue.
Speaker 3:If I've now want to get part of the action and I want AI bots doing my job for me, then what's your purpose? So you have first line. That's automated. Second line is becoming automated. So you have three lines of. So who's actually checking all of these machines? You have three lines of machines.
Speaker 3:If you're going to automate your job away, well, why can't first lines automation or second lines automation do the same thing as yours? Why do you need multiple bots? The whole purpose is having someone objectively saying does that make? Are they doing the right thing? This is something that it audit, as really I think they're feeling left out and they want to get in on that. And there's a big issue of, like you maybe use AI based systems to help you better find anomalies and better find issues, that's okay.
Speaker 3:But being like, I need to learn how to program, I need to learn how to use these tools, I want to apply these tools in my audit department. I see that a lot. I see that almost every single audit conference I go to and a lot of people are talking about that way and that's, I think, completely wrong. If you want to be doing that, great, become a data scientist and work in first line, but you can't be automating audit, and that's a major issue, I think, and that's a big concern. If you see auditors be automating their job away, then there's no one actually auditing anymore, and that's a major concern of mine in the audit industry in general right now of feeling like they're missing out, so they're trying to go in to do that.
Speaker 3:So I don't think you should, as an auditor, learn how to program. You can. You don't understand what's going on, know what's hype and what's not, but you need to be the objective high level. It's hard to be the 15th person code reviewing. You're not going to provide a valuable insight. However, why are we even doing this in the first place? How does a connect with B? That's where the important part of audit can come in.
Speaker 2:Yeah, I think, I think that that's really, that's really powerful. And you know, auditors are feeling the pressure everyone else is feeling, but what can they do? Like today, like what's in practical they can start doing, to really start aligning themselves with what auditors need to accomplish and what and how they can be truly helpful, rather than being, like you're saying, like the fifth data science in the pipeline.
Speaker 3:Well, that's what we're really focusing and honing back on to what are the core fundamentals of auditing and what is the core thing you're supposed to be doing. It's usually sample based testing or understanding the systems, doing walkthroughs of what is this supposed to be doing and why. And how do you know? What controls do you have in place? How do we know they're working effectively? Going back to those, that's what key auditing doctrine of what you do as an auditor asking those questions and why did you do this?
Speaker 3:Data scientist? Why are you automating this process? Is that, does that make sense or not? But just be the own up to like hey, I don't know what you guys are talking about, but I can tell if you're just bsing me, if you actually know what's happening. But why are we doing this? Sometimes we're so in the academic researcher data scientists were so deep in the tech that we, for the loose side of what we're doing and why, so really honing back into what is this doing for the business, is the risk being mitigated for the business? That's really what audit should be working at. So of course, you need to understand critically what are these technologies capable of and not, and where are there risks areas. That's what auditors should be focusing on learning, not how to program, in my humble opinion.
Speaker 1:And now I asked before in the data role, in the data and the research roles. I want to ask you again here objectivity of an auditor? It sounds like we're circling around that 100% and auditors need to be objective.
Speaker 3:So, like you don't have to be as a data scientist, you know objective review by somebody else, something, but you don't need to be objective from the data, like your point of if you're a domain expert in manufacturing, you don't need to be objective. Like you should be subject, you should, you need to. You know every professional needs to try and look at their problems objectively right, but you need to know in depth what that data is and how it was generated and things like that. Right. As an auditor, you still need to have domain knowledge, but you are have to be super objective away from the problem. You have no stake in the game of oh, I got to make sure I approve this, or like a whole thing about auditing.
Speaker 3:One of the tenants of auditing in general is that objectivity from the outcome is you're trying to objectively fresh set of eyes and this makes sense as it is the company adequately mitigated the risks. As an auditor, that's what you should be doing. So objectivity is the name of the game. Data science first line yeah, it's not you. Second line should be validating you. They're the objective ones. First line, you are subjective and that shouldn't be a real concern at that level.
Speaker 1:So, as we've bottled up, from the model builders, the foundation, to the marketers, the messaging, the product, and out to the audit, out to risk and audit, now wrap this up for the executives. What is their key role? What do we wish from them? What do they wish from these roles?
Speaker 3:I can always go on a little bit of rant on this one as well, but I think executives so much trying to one ups themselves keeping up with the Joneses I think they said this. I got a kind of, of course, some generalizing not everybody's like this. Executives need to know when to try and learn the discernment of when do I need to push my people to find innovative solutions and when do I need to trust the professionals I have and, if I might, I have some. I poached MIT, stanford, ph, mit, linguistic, nlp researchers and they're all telling me we can't do this. But Gartner, I was on a phone with their BA and English analyst and they said that everybody's doing this already. Well, who should I listen to? I've coached these MIT researchers and they're telling me that's not possible. But Gartner said it's possible. I should listen to my people. You know, like that kind of thing was, like no wind, to how to get the signal from the noise.
Speaker 1:I actually have a hot take on that, but I want to make sure that you know, sid, you get your chance on. It's just some advisement to the executives who are really trying to navigate what the foundations of AI can mean to their business as they're trying to sustain with newer technologies.
Speaker 2:Yeah, I mean as always, Andrew, at least, with almost nothing to say, but I agree that this is very similar to our domain expertise conversation. Right, you should talk to people that are in your organization that do this work. If you want to have a grounded, real understanding of the timelines and the possibilities and the use cases that these technologies pose, you should talk to the people that work for you, rather than relying on the media landscape. You might find that, oh, this is very easy for our team. We already have that. That's a fun conversation. Or, oh, no, we can't do this and actually we thought about this and there's a reason we haven't done this right. So it's truing back to your technical teams and really understanding why things haven't been done. And if something hasn't been done that should have been done, are you doing it from the right place?
Speaker 3:I think that's well said Key thing that in some areas, executives are like we want to be differentiated and have a moat, but in other areas they want to fog, they want to do keeping up with the Joneses. So I think it's a key area. When it comes to technology, what's the core reason? The fundamentals of your business and the fundamentals of that competitive moat that your company has. What is it that you guys are doing? If you are, we are an AI-based company just trying to have new solutions, Of course you're spending as much money possible pushing that. But if you're a manufacturing company that makes Lego, for instance, like OK, well, who cares what IBM is doing with AI? It doesn't matter to me because that's not the focus of my business. So calibrating that, I think, might help as well, and, of course, there's some executives that do a great job of this. It's just part of what we hear in the media and there are definitely people that are doing this chasing.
Speaker 1:Now and to your point in that same example. It's listening to your customers. What do you do for your customers? Well, how will your customers benefit? And taking that as a North Star as well, I think actually, if I could put it back from the organization up to the executives, when the majority of your organization can't be in front of the customers, that organization really depends on you to really have that focus. That North Star is like at the end of the day, how is it going to benefit them and not only retain customers but attract new ones? Because at the end of the day, you're in business for customers, otherwise I don't know how you get paid. I agree.
Speaker 3:And I think a good way to close this out for and then, of course, susan and Sid, final thoughts, for AI isn't anything else in life, and I think this is the crux of the issue is people always want to take the easy road and there's some, like magical fate, greener pasture on the other side or whatever. I don't know if you guys are familiar with Ryan Holiday. It's written some really good books. One of them is called Obstacle is the Way, which is about like Stoicism and things, which is kind of an ancient philosophy thing, but essentially the hard way is usually the best way to do something. Same with, like Navy SEALs have a thing that only Easy Day was yesterday. Like the hard path, the path less chosen, the hard yards, is usually where the most rewarding thing is.
Speaker 3:If you're chasing the lemmings of whatever everybody else is saying AI can do for you, well, your product probably not going to have high quality. A lot of your business won't be. So it's like really determining and understanding. It's back to the fundamentals. The fundamentals and whatever discipline you are, if you're a CEO trying to do sales, if you're a marketer, if you're an auditor, what are the fundamentals? Like we talked about with auditing, objectivity and being that last safety, stop right. What are the fundamentals of what you're doing and how do you make sure you're doing the right process? Most people treat AI specifically, this LLM thing, these magical things that will make the world easier. There is no easy answers and I think the sooner that we all realize there is no easy button, the better will be. And AI is not the easy button.
Speaker 1:Yeah, very well put and I do believe that, like I've said before, I do believe that this is the year that we're actually going to be closer probably the closest to figuring out what that is you mentioned outside is the way, is a really good resource for really establishing some ground on picking a direction. I think Sid has some Sid and we've been compiling some other educational resources.
Speaker 2:As an outsider looking in, yeah, so I mean, this is my, I guess my final piece of general advice, and this is for everyone, but also people that are outside, people that do nothing with AI, people that work tangentially, are analysts or recruiters. What can we, what can we all do, developing our sense of media literacy? Reading these articles, what is hype? What are the incentives of the authors? Is there something that they're going to gain from this, or does this seem like it's really about the research and the possibilities and the use cases? So development media literacy is a big part about being in the space and seeing who is doing it, the right one who's just trying to do whatever an else is doing with a new coat of paint. I'm sure Susan will leave some great publications that Andrew and I have worked on. We've been talking about this for a long time. We like to think that we keep them generally easy to read For a non-technical audience.
Speaker 2:It could be a little tough, but I think that these are things you could push through with you know a couple of Wikipedia's and if you're especially ambitious and you're an outsider but you want to get inside, I think the easiest place to start is really just to try one of these Coursera courses. The Andrew and G1 is really popular. Mit gives free courses, stanford gives free courses. You can have the same knowledge that everyone else has, maybe without the accreditation, but you'll have the same knowledge base. And then I would challenge you then to try and watch some YouTube videos, which are really boring, like 100,000 views or less. Channels that are really just talking about the subject, because, like, I'm passionate about this and I want to teach you about it, not channels that are like here's how you're going to make $300 a day running a chat GPT model People that really want to educate you. And that's what I would challenge a really ambitious listener to do trying to get inside.
Speaker 1:I like that point because there are a lot of people out there who have really good thoughts and I'll take the challenge to go and find some of these, to put them out there. On some people who are really good, they may not have the publicity that the people who want you to make money off of their course do, but are good just the same. If you have responses or you have questions, this was a deep episode, unlike the technology. We went into the people aspect and the teaming aspect of things. So if you have questions, our homepage for the podcast is at the bottom of each episode and we have a submission form there. Now you can submit your comments and questions and we will. Either we will get back to you and if we have a good exchange, we will include it in a future episode. So thank you for joining. We'll see you next time.