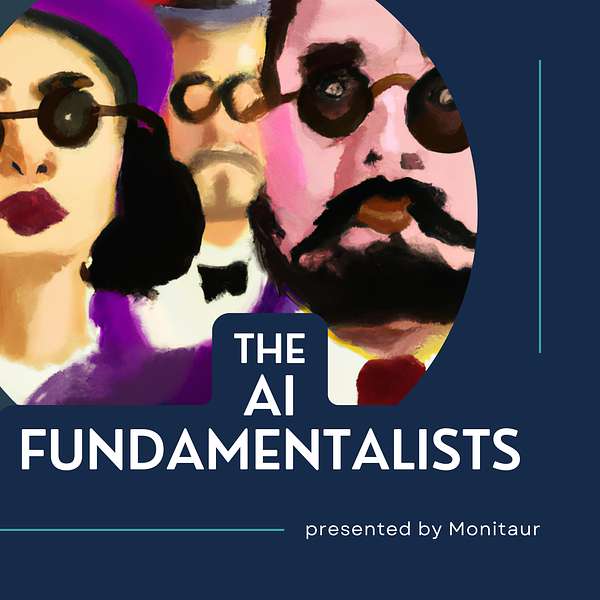
The AI Fundamentalists
A podcast about the fundamentals of safe and resilient modeling systems behind the AI that impacts our lives and our businesses.
The AI Fundamentalists
Exploring the NIST AI Risk Management Framework (RMF) with Patrick Hall
Join us as we chat with Patrick Hall, Principal Scientist at Hallresearch.ai and Assistant Professor at George Washington University. He shares his insights on the current state of AI, its limitations, and the potential risks associated with it. The conversation also touched on the importance of responsible AI, the role of the National Institute of Standards and Technology (NIST) AI Risk Management Framework (RMF) in adoption, and the implications of using generative AI in decision-making.
Show notes
Governance, model explainability, and high-risk applications 00:00:03
- Intro to Patrick
- His latest book: Machine Learning for High-Risk Applications: Approaches to Responsible AI (2023)
The benefits of NIST AI Risk Management Framework 00:04:01
- Does not have a profit motive, which avoids the potential for conflicts of interest when providing guidance on responsible AI.
- Solicits, adjudicates, and incorporates feedback from the public and other stakeholders.
- NIST is not law, however it's recommendations set companies up for outcome-based reviews by regulators.
Accountability challenges in "blame-free" cultures 00:10:24
- Cites these cultures have the hardest time with the framework's recommendations
- Practices like documentation and fair model reviews need accountability and objectivity
- If everyone's responsible, no one's responsible.
The value of explainable models vs black-box models 00:15:00
- Concerns about replacing explainable models with LLMs for LLM's sake
- Why generative AI is bad for decision-making
AI and its impact on students 00:21:49
- Students are more indicative of where the hype and market is today
- Teaching them how to work through the best model for the best job despite the hype
AI incidents and contextual failures 00:26:17
- AI Incident Database
- AI, as it currently stands, is a memorizing and calculating technology. It lacks the ability to incorporate real-world context.
- McDonald's AI Drive-Thru debacle is a warning to us all
Generative AI and homogenization problems 00:34:30
Recommended resources from Patrick:
- Ed Zitron “Better Offline”
- NIST ARIA
- AI Safety Is a Narrative Problem
What did you think? Let us know.
Do you have a question or a discussion topic for the AI Fundamentalists? Connect with them to comment on your favorite topics:
- LinkedIn - Episode summaries, shares of cited articles, and more.
- YouTube - Was it something that we said? Good. Share your favorite quotes.
- Visit our page - see past episodes and submit your feedback! It continues to inspire future episodes.
The AI Fundamentalists a podcast about the fundamentals of safe and resilient modeling systems behind the AI that impacts our lives and our businesses. Here are your hosts, andrew Clark and Sid Mungalik. Hello everybody, welcome to another episode of the AI Fundamentalists. Today's guest we have Patrick Hall, the Principal Scientist at hallresearchai. He's also an Assistant Professor of Decision Sciences at George Washington University School of Business and serves on the Board of Directors for the AI Incident Database. He is also an accomplished author. His latest book, machine Learning for High-Risk Applications, was published just late last year. With that entire and stellar resume, patrick, welcome to the show.
Speaker 2:Thank you. Thank you for that kind introduction. Happy to be here.
Speaker 3:Yeah, and we're glad to have you here too. So one question we like to ask all of our authors because it's always a good question is you know, what did you learn writing this book about machine learning for high risk applications?
Speaker 2:I think for me, the learning actually came after the book. But for me, the book was a chance to kind of reflect and put together a lot of work that I had done across governance, across explainable models and explainability, across bias, testing and model validation and security, and kind of get it all under one roof and, and you know that that was a productive and fulfilling exercise. But I think that you know, the learning really came after the book, or at least you know, after I was done with the book. I, the author team, finished up the book in April 2023. And you know that that's when the generative AI hype really really started to hit. And you know there was this there was definitely a sinking feeling of oh no, you know, it's like we, we just put this sort of capstone work together and now, you know, is it going to matter in this sort of like flood of hype around generative AI? And I would say I would say that you know, the jury is still out on that. The jury is still out on that, for better or worse.
Speaker 3:Yeah, and I think this really gets at the sense that, like responsibly AI is not just one umbrella topic. Right, it's a lot of small topics. In every way that we do AI actually generates different ways that we can do it responsibly. But do you feel like there was maybe a strong central theme in what makes these responsible AI patterns possible?
Speaker 2:Yeah, yeah, and I think that's why you know this notion about like is this work going to stick around during the time of generative AI Like? To me, it's so clear that it should. But because that theme is that, that unifying theme is outcomes focused. So right, nist AI risk management framework might might call it socio-technical, whether you, whether you like that term or not I do, but some people don't, you know. You might call it outcomes focused, right, so you know it doesn't really matter. You know for users if a system does poorly on some static test data set or some, you know, prompting benchmark. What really matters for users is how the system behaves in the real world, and I think that the book really does focus on that and I'm hopeful that that message will stay relevant despite the kind of crushing generative AI hype wave.
Speaker 3:And it seems like in a lot of your work and research that you've done a lot to back up the NIST AI risk management framework. So I guess what specifically about NIST really encourages you to speak highly of it, to promote it to you know, use it as like almost like a go-to starting point for most people working in this field.
Speaker 2:I think there's a couple of things about NIST that make it stand out, but none of it is special to AI. So you know NIST has pursued many technical frameworks, right. Probably the most prominent in technology are the cybersecurity framework, which is, you know, going on roughly 20 years old. The data privacy framework, which is going on roughly 10 years old, and the AI framework, which is going on roughly 10 years old, and the AI framework, which is going on roughly one year old, right. So they have about a decade separating these long-term kind of risk management programs that you know they impact different aspects of technology and you know what is what's. You know I don't represent NIST, I'm not a government official, I'm a, I'm a grantee who conducts research supporting the AI RMF. But from my perspective, what's what's so important about NIST is is two things they don't have a profit motive. So almost everybody else out there telling you something about responsible AI or how their systems are responsible would also like to sell you that. And I think that if you think back to a time when AI wasn't so dominated by corporate interest, you might be a little bit suspect if someone wants to sell you something and oh, by the way, they're telling you it's really good, right? Traditionally we would just call that a conflict of interest, but you know we don't talk about that in AI today. So so NIST doesn't have this sort of profit motive conflict of interest that almost everybody else who's who's talking about guidance has.
Speaker 2:And then to NIST in my experience is really truly a democratic organization. It's not like the people at NIST are smarter than other people, but I do think what they're good at, what they are better at other people, is these democratic public comment exercises right. When NIST puts forward a piece of guidance, it's been reviewed by almost every federal government agency. There's been a comment period where every company or every private individual was able to comment. And then I can assure you that NIST scientists and people like me sit on long, boring phone calls where we just adjudicate all of these comments. You know. So not only is there the institutional knowledge of NIST, like it's really drawing on the hive mind of the American public, and you know American industry to come up with really high quality products that aren't driven by a profit motive. So I would say that to me is what's special about NIST? Not that it's perfect.
Speaker 1:So can I ask a side question? Yeah, when we're thinking about NIST. Not that it's perfect, so can I ask a side question? Yeah, when we're thinking about NIST? One of yesterday's headlines in TechCrunch was the FTC is investigating how companies are using AI based pricing on consumer behavior, and when I saw the headline, I posted it to our group know, I posted it to our group to saying like hey, here's another outcomes focused review on ai and these are some pretty big names and companies that were using consumer behavior to price adjust.
Speaker 1:Yep, um what in in your discussion about what you just said about nist? What would you think of that? How would you respond to that?
Speaker 2:Well, so I think there's two things. So first, yeah, prices and law and violating laws and, in, you know, regulatory enforcement actions. Those are some of the. You know, what do people care? What do really people care about? Money and laws, okay, so. So actually, when we're evaluating ai systems, it would be good to think about money and laws and not um benchmark scores based off other broken ml classifiers and other static test. You know data metrics, so so one. I think that's exactly what we mean when we say outcomes focused. Now, nist is always quick to say they are not a regulator. You know the AI RMF is not regulation. So, you know, I think the law enforcement aspect is apart from the sort of NIST guidance. But I think that law and money are two of the very clear kinds of socio-technical things that we might think about when we want to build good AI systems and that I think are generally ignored in a lot of product design.
Speaker 4:The NIST process. I love how you guys have worked through that and we were contributors to each of the rounds of the NIST risk management framework and really appreciated how you opened that up to other companies and things to have influence on the final.
Speaker 3:So working through the risk management framework right it's. It's a nice hefty document. It's got a lot of suggestions in there. It's got a lot of guidelines in there. Do you feel like organizations that want to start embracing these ideas and this framework and start building the way around it Do they? Do you feel like it's going to be a wholesale shift for organizations to adopt these types of methods, or are there specific pieces of it that you think are easy to start picking up today?
Speaker 2:That's a great question and I think you framed it correctly. So essentially it just depends. As you're probably aware, like in the consumer finance vertical, big US banks, you know, tend to have pretty mature model risk management practices and you know the model the AIRMF in many ways is based on those model risk management practices risk management practices. So I would say that, you know, just for example, for a large US bank to adopt the AIRMF, while it would be complicated because of their existing processes and policies and the oversight in terms of actually changing what they're doing with AI or machine learning, it's probably pretty minimal, you know. Another example of adherence being minimal would be, you know, of course, the AIRMF says you should be using explainable models. You know, and many, many people do use explainable models sometimes, whether they know it or not, and so I would say that's an example where it would be easy to follow this guidance.
Speaker 2:I think places where it's difficult to follow this guidance are the parts of the guidance that strike at negative aspects of sort of AI and data science culture. Right, documenting your models, signing your work, changing your culture so that there's human accountability for AI outcomes Right. These are things that many tech organizations just honestly appear allergic to, and I think it will be very difficult and take a long time for them to. You know, experience that culture change that switches from you know. You know experience that culture change that switches from you know.
Speaker 2:I'll just pick on Google. Google's quote unquote blame free culture you can't have gold, you can't have governance in a blame free culture. It's not possible, or I mean you can't have what amounts to real governance. In a blame free culture. You can talk about governance, you can do marketing on your governance, but but you don't have real governance unless someone is blamed when things go wrong. That's a basic aspect of governance, and so I think those types of cultural changes will be the hardest pill to swallow. And that's not going to come from the AIRMF, right, that's going to come from FTC enforcement or SEC enforcement. There's going to come from FTC enforcement or SEC enforcement.
Speaker 1:There's going to have to be a stick involved, and NIST is simply not that stick. Yeah, I feel like we could do a whole show on the nuances of blame-free culture versus productive, like you know, building better technology yeah.
Speaker 2:I'm not sure that a blame-free culture and better technology for consumers are compatible.
Speaker 4:I haven't thought about it that way. That's a great. Governance really has to be who's responsible for something, and if it didn't get done, it didn't get done. That's a yeah. I learned that it has to be accountability and, in fact, that's a yeah, I learned that there has to be.
Speaker 2:Accountability and in fact that's a main driver of documentation is that you put your name on your work so that when something goes wrong we know who to call right. And yeah, so the accountability piece is going to be difficult for cultures that have eschewed accountability to get used to, and it won't be the NIST AI RMF that makes them do that. But the AI RMF could be part of a much larger kind of mechanism that slowly changes the culture.
Speaker 4:Agreed. I think a part of that as well and one of the issues we see with a lot of people is that lack of objective validation and independent processes of those lines of defense right like it's a lot of tech companies like to be, like we, we're all a team, we're doing it together, we're figuring out this thing, but you have to have that objective somebody because no matter how great your team is, you'll group think right and like having somebody having that explicit responsibility and someone signed off on it. Like that, no matter how great your team is, you won't find all the errors and possible like edge cases students are listening, and I'm sure they're not, but I do have a.
Speaker 2:I have an exam question, a short answer exam question that says if everyone is responsible, then who is actually responsible? Right, and I feel confident enough to ask that question on exam because, very clearly, the answer is no one. Right, if everyone is responsible, then no one is responsible.
Speaker 2:And you know, I again like it's going to be hard to change that culture in in. You know, again like it's going to be hard to change that culture in. You know, move fast, break things, tech land. And then I want to be clear that you know if we're making some kind of non-serious you know, I don't know. You know some kind of ads for video games on people's phones like go fast and break things right. It's just when we get into medicine and hiring and managing elections and managing critical infrastructure, like please don't go fast and break things.
Speaker 3:And as we think a little bit about company culture and adjusting our expectations around, like you know, what's the difference between building fast and working in these regulated markets? We see a lot of teams and companies out there that are saying, like, well, we had this explainable model, but let's just do it the LLM way. Now, right, let's use that large language model. What does that conversation look like? And how might you convince the team? Hey, you know, we have these great existing models that work and we can explain their decisions. Why do we need to use LLM?
Speaker 2:Did you guys see out of Yandex just recently this tab red benchmark? So there's been a I don't know I picked it up there's been a couple of new benchmarks of models on tabular data, I don't know, in past months and years and for whatever reason, this, this new one called tabred, jumped out to me. Um, in fact, if you hear my gpu fans going, I'm I'm rerunning this, uh, tabred, it's going to take a lot longer on my uh little dual 2080s than on the a100s that they were using. But I mean, I'm just going to rerun the benchmark and put explainable models into it and see where they fall, and I suspect they'll fall right under the best performing black box models. But we'll see. I'll share those results. So one I think you know it's, there is a use of just kind of like look on these eight data sets. The explainable models perform just as well on our static test data sets or, you know, within a margin of error, as the unexplainable model.
Speaker 2:So I think there's two really crucial things if you're trying to get people to adopt explainable models. One is you have to have that outcomes focus, because if you don't have an outcomes focus, you're going to be like, well, the black box has a better AUC, you know, in the fourth or fifth decimal. So you know we have to use that. And then you have to acknowledge variance, right, you have to acknowledge it kills me, I don't. You know my again. You know, like, my first data science interview question is always what is variance? And 80% of data scientists cannot answer that question. They sit there like a deer in the headlights and that is a failure of our educational system, if nothing else.
Speaker 2:So you know, if you're looking at a leaderboard and one model is ahead of another model by some tiny number and there's no margins of error, like, please stop back away, engaging in scientific, you aren't. You aren't engaging in science, right, like you're just playing with numbers. And I think, if we're honest, right, a lot of the fun, cool stuff that data scientists do at work is just playing with numbers. And you know, and and in fact the the NIST AI bias standard, sp 1270, gets into that and go so far as to say that that you know a lot of what we do in AI is cargo cult. Science has the trapping that goes back to Richard Feynman, has the trappings and appearance of science, but not the core of science, and unfortunately. I do think that's true. So if we can be more outcomes focused, if we can acknowledge variance, then I think using explainable models becomes a lot bigger. You know a lot easier of an argument to make, but those are hard arguments to make inside of large organizations, undoubtedly Love all these quotes.
Speaker 4:These are great. I couldn't agree more. Thank you so much for these great insights for these great insights Very welcome.
Speaker 2:I mean, and I will say, you know, as like, how do we get people to not use generative AI for decision making, which it is not designed for? I don't know. My, you know, I'll tell you what doesn't work. Making factual arguments about the design and validity of systems. That doesn't work. And in fact, I'd say for anybody listening in, like, if you're about the design and validity of systems, that doesn't work, and in fact I'd say for anybody listening in, like, if you're doing that inside your organization, you may not be doing much more than putting your job at risk right Now.
Speaker 2:You're right. You're right, you know check mark for you, but you know it's not what people wanna hear right now and I think, unfortunately, we're in this. We're gonna deal with a couple years where generative AI is too big to fail, right, like it's not really going to work, it's not really going to make any money, but the movers in the market you know both in terms of on the venture capital, a big tech side, everybody's way too invested in it. It's simply not going to go away and it's just, yeah, I would say, the generative the way I describe generative AI these days is too big to fail and we're going to be stuck dealing with it for a while. So on the risk management side it's almost sort of good news because there's going to be a lot of risk management work to do. But I don't think it's necessarily good news for the field of AI, for the sort of commercial practice of AI.
Speaker 3:Yeah, I think we do feel a lot of that weight where the large tech companies have invested so much money and they're looking at 10, 15 year time horizons before profits are here. So they actually can't give up right. They're in this game for the very long haul.
Speaker 2:Yes, that's what I'm saying. So so I, I think it's pretty clear that the original, you know, set of promises like, oh, it's going to be your doctor, it's going to be your lawyer, it's going to be, you know, poor people's doctors and places where there aren't doctors, you know, I, I hope it's clear to almost everyone that that you know, those promises aren't going to come true with this current generation of technology. But, as we've been discussing, it's too much of a business proposition at this point. It's simply going to be pushed on us. It's going to be pushed on us. So, yeah, I mean, I expect to spend the next five or 10 years teaching students not to do this. And, you know, dealing with the fallout in corporate practice of people using systems that are designed for content generation. You know people using those for decision making and, in particular, high stakes decision making, where they should have been using an explainable classifier from the book.
Speaker 3:Yeah, yeah, absolutely. So I guess, like you know, when you're working with these students, you know what are. What are some very obvious gaps in their beliefs about responsibly. And I ask this because you know, I think that students almost model the market, in the sense that they're probably interested in these topics but they're not yet invested right. They've only started to dip their toes in.
Speaker 2:With students. I think that they are impacted by AI hype, right, and then I think so, not only are they impacted by AI hype, they're impacted by AI like much more than me. Like I make a very serious attempt not to be impacted by AI hype. They're they're impacted by AI like much more than me. Like I make it, I make a very serious attempt not to be impacted by AI. Not I'm sure my face is being run through some filter or something right now, you know, et cetera, but like my, my smartphone is locked in my desk drawer, I'm barely on social media, and and so I think that that I think that students are actually, you know, just because of where they are in their lives, they're so online, they're actually impacted by AI a lot, and negatively impacted. I think that they generally have some understanding that it's not great that every single one of my social interactions is being commercialized. I think that's a pretty easy thing for people to understand how that could go wrong, but I think that they they lack the vocabulary to discuss it, right, they just know it feels icky, something like that. They don't know, they don't know how to discuss it in terms of recourse or data privacy violations or intervenability, and so I think I do really enjoy giving them that, that vocabulary to enable them to sort of put a finer point on these experiences they're having.
Speaker 2:But at the same time, you know, I do feel concerned that that, with the AI market, market the way it is, like I'm not sure I'm helping them in their jobs right. Like I worry that, you know, I put in their head all these big ideas that, like you know, ai shouldn't be broken, and then they're going to get their first data science job and that's going to say rush out this broken model as fast as possible, and then I won't have done them any favors. Um, another so. So another thing with students, though, is students have to write a lot of assignments, and generative ai is actually pretty good at that right, especially those those kind of first and second year pro. You know what are so. So an assignment that I I used to give and I had to stop giving is just what are the pros and cons of a large company adopting machine learning right, and ChatGPT can do a really great job at that particular essay.
Speaker 2:So I would say, like students may get the most out of these tools. In some ways, students may get the most out of these tools in some ways. And another thing I have to bring up is English fluency. Right, that generative AI is really helpful for, you know, smart students who want to express their ideas but they don't have the English vocabulary to do it. And again, I think like generative AI can be super helpful there to do it. And again, I think like generative AI can be super helpful there. So I think students, like the rest of us, have a pretty nuanced experience with AI and these generative AI tools that it's hard to pin down. So I'll just you know, I'll leave those comments as kind of a flavor of what I think they're experiencing.
Speaker 3:Yeah, I think that's a great answer and I think that you know we are learning a little bit now about what is the language, of how we want to talk about these issues right, because we only talk about them in you know, essentially, yeah, you know Ick, you know AI bad, but we're not really getting at the pieces of what really disturbs us about this and you know surveillance parts of it and the decision making parts of it.
Speaker 3:So I think there is a lot of room for students and the market in general to learn a little bit about how we want to talk about this, because that'll give us the tools to actually work through these issues.
Speaker 2:Yeah, and it's important to talk about it. Like I said, I've really cut off my exposure to social media, but I did a little LinkedIn post actually for my students yesterday, so I was on LinkedIn more than I usually am. That's the only social network I'm really on and I was actually surprised to see, scrolling through my feed, the number of people who are saying the kind of things that I'm saying now, which a year and a half ago like nobody.
Speaker 2:That would just have been career suicide. I was saying them I committed career suicide. If you want to run a small company and be an assistant professor and be on a volunteer board, you know be more honest about AI. But no, I was sort of comforted to see that people have started talking about this more, which I think will be an important part of changing the culture around it.
Speaker 3:Absolutely, and so I guess now for like a bit of a pivot, since I think this will be, you know, fun for the listeners and you know probably, fun for you too. We'd love to hear more about these AI incidents, right? You helped maintain this AI incident database. What's an incident in recent history that really stood out to you?
Speaker 2:Well, an incident that came up on a client call earlier today because a big company thinking about adopting generative AI for interacting with their consumers I think a lot of companies could learn from. I believe it's incident 475, which is the debacle with the McDonald's drive-thrus. So the debacles with using AI in McDonald's drive-thrus, and I haven't had a chance to verify this. But what the client was telling me today is, you know she saw that incident and now you know she's reading that McDonald's is actually shutting down the AI programs in the drive-thrus. So I haven't, like I said, I haven't been able to verify that, but we were tracking, like, a large amount of customer dissatisfaction with AI in the drive-thrus, which is actually a pretty constrained application. Okay, like, like, there's a limited number of things on that menu and there's a limited number of of sort of possible responses, and I think it's striking that it could fail in in that kind of but not surprising to me, uh, that it that it could fail in that in that kind of scenario. But but, um, you know, maybe I should google really quick and see if they are actually taking it down, but that's what I heard on my last call.
Speaker 2:Um, another, um, another incident that I bring up all the time that I think gets at just the basic limitations of today's intelligent systems is um, when California, you know, say I, this was, this was older, maybe 2000,. Maybe five years ago. Let's say um 2000,. Maybe five years ago, let's say, mapping apps like Waze were sending drivers in California into forest fires because there was no traffic in the forest fire and nobody, you know, I don't think anybody was hurt. I think you know the roads were closed, police were stopping people before they got to the fire. Anybody was hurt. I think you know the roads were closed, police were stopping people before they got to the fire. But I think that that gets to the inability of our current automated decision making systems. Whether it's optimization, you know route optimization and mapping. Whether it's generative AI, whether it's signal processing, descriptive, you know predictive AI. These are memorizing and calculating technologies. They are not reasoning and judging technologies and they lack the ability to incorporate real world context.
Speaker 2:You know, and I hear people talk about context all the time with language models, but and what I try to reorient them to is like, look, you are talking about context in the digital data world. I am talking about context in this real, physical world that, by the way, we still all have to live in and live together in, and I don't know. That point just never seems to go through. Like I'm a little worried about people mixing up digital context and real-world context, because that's kind of scary.
Speaker 2:But yeah, current automated systems are memorizing and calculating technologies with no ability to handle real-world context, and that means that if the road changes or the situation around you changes, your mapping app does not know and it will send you into a dangerous situation. And there's nothing about generative AI or predictive analytics or signal processing. Mapping app does not know and it will send you into a dangerous situation. And there's nothing about generative AI or predictive analytics or signal processing. That's any different right. And so I think the way sending drivers into forest fires because there's no traffic in the forest fire is like the perfect example of the failings of our current generation of technology.
Speaker 3:Absolutely, and I'll even double down for you here on context is missing. So in the NLP world, we talk about like oh, we need user context. And so user context is like well, what did you say yesterday? What are your Facebook posts? What is your Twitter bio? Right, that's the extent to which we talk about extended context. But even digital context is so much larger than that. Right, digital context is like what kind of person is this? What are their fundamental beliefs? Are they at risk for certain behaviors? These are absolutely not captured in these models. You know, people might claim that they're captured implicitly, but that's not. You know, a decision factor making at all, and that's you know. Even going beyond what's in the real world, they're not even working with what they have now. Right, the scope that they work in is much smaller.
Speaker 2:Right, and I mean just that makes me think of there's a number of incidents about predictive policing and use of AI in policing, particularly to identify people. And you know the New Yorker's article, if you have time and you can stomach the New Yorker, their article on how police behave when they're using these AI technologies is is really astounding. Right, we haven't gotten into human AI configurations, right? So, again, you know when I, you know Greg Brockman, evals are often all you need. I mean I guess he did say often, but but one thing that evals are not going to tell you is about human reactions in the real, physical world to digital technologies. And and I imagine that, like the McDonald's incident that we're talking about, has a lot to do with that but the New Yorker reporting on how police, you know, smart, experienced police officers essentially shut their brains off when a computer tells them that someone commits a crime, I mean, it's just, it's just freaky, it's just absolutely freaky. You know, in many, in many, many of these sort of wrongful AI, wrongful identity cases, they're going out and arresting people who are 30 years older than the person in the picture because the the, you know, the facial recognition system, which wasn't properly validated, which, by the way, there's a good.
Speaker 2:There's a good quote about how it's. It's easier for it's easier to buy law enforcement AI technologies in many cases than it is easier to buy a snow cloud, like if you're a county government. It's easier to buy fancy ai technology for your law enforcement than it is for you to buy a snow cloud, um and and so you know, there are all these real world issues. This is context, right, like regulation money, uh, people's ages, fires. This is this is what I mean by context. I don't mean a, you know, 2048 token context window. That's not what I mean personally.
Speaker 1:Patrick, I have a question about the with your students. You mentioned that, you know, and even when we were like preparing for this episode you mentioned, you know you really do encourage that they should be using gen ai for all the reasons, for all the good and bad reasons, that is, it exists that you have to be experienced with what you're getting out of it, um, but also with regard to language, like reducing language barriers, have you seen any studies or anything with regard to the reverse of that, like if you're taking in broken English, that might come from that?
Speaker 2:Sure, yeah, that's a very good question and one of those great human AI configuration issues that we'll have to deal with. To be honest, I haven't heard, I don't know enough about the part about sort of students kind of learning wrong English or you know incorrect patterns in any language from a chatbot. But what I do worry about and again this all comes from work at NIST is homogenization. Right, these language models, they're big regressions, right. So they predict a conditional, mean, they're predicting a mean response, so they're predicting essentially like the most normal response to any question. And so I do worry about, you know, homogenization of ideas, homogenization of cultural representation. And then there's another aspect, you know, and this is all just research, I don't think we know how any of this plays out, but you know there's a lot of research that says if you train a language model on synthetic, synthetically generated model from data from other language models, you know the performance of language models drops off quite significantly and within, say, like 10 generations, you have full, what they call model collapse, and so so all of those get at this issue of homogenized content. But what's tricky here is from a cultural perspective, from a creative perspective, and when people say these models are creative. I wish that they would get educated on that topic, even though sometimes the CEOs of the companies that make them say that kind of thing, they're not creative, they're just generating average responses. So this issue of homogenization, it's bad for creativity, it's bad for art, it's bad for, you know, people of different cultures, people with different dialects, but who it could be really good for is big companies that want to speak with one voice, and so all of this stuff is.
Speaker 2:I think that the way to sum this up is all of these issues that we've been talking about are way too complicated for technicians to figure out on their own right, like that's hopeless. Ai has become so important, perhaps too big to fail, that it's simply causing all these social and environmental issues that are going to require input from a broad group of stakeholders and not just an insular group of technicians. You know, working 24 hours a day. That's not going to solve any of these problems. So it was a great question, susan. I don't think I answered your angle, but this notion of homogenization of content has a lot of cons but also a few pros, and it's just complicated.
Speaker 3:And I think you know I couldn't write what we said in our synthetic data episode, which is, like you know, as these models eat what they output and they do that over and, over and over again the variance of these machines it just plummets, and we're maybe already starting to see this with some of these image models that it's like, well, you're not going to let us use copyrighted data, Then we're just going to use our own outputs, and you just start seeing the same thing over and, over and over again.
Speaker 2:Yeah, yeah, and we didn't talk about intellectual property.
Speaker 2:But I think that you know I'm not an attorney that's gonna have to be worked out in the courts, but I think you know I don't.
Speaker 2:If I was a big company thinking about adopting generative ai, um intellectual property violations and and you know potential liabilities and fallouts around that, that that would be on the top of my mind and certainly not, you know, skynet and this stuff telling people how to make biological weapons or performing impossible hacks. You know, I think that it's the real burning risk here with generative AI, in my opinion, is intellectual property infringement, and I'm not a lawyer or a judge, so I don't know, I couldn't, I couldn't tell you how that's going to play out, but I think that there are large actors who are incentivized to sweep intellectual property issues under the rug and perhaps flag, you know, less realistic issues like Skynet and these things telling people how to make chemical and nuclear weapons that I'm just not sure that they're capable of doing that. So it's all very complicated and outcomes focus right Outcomes focus. If you're just living in test data world, it's very easy not to think about these things.
Speaker 4:Definitely. Well, it really just comes back to what we talk about on the podcast a lot here which is like the fundamentals matter. There's no easy button for anything. Everybody thought janae, I was easy button. Everything comes back to really good governance, solid blocking and tackling set responsibilities. Yeah, doing things the hard way yeah, yeah, I think you know.
Speaker 2:Maybe the last thing I'll say is there's a statistics professor at one of the big Midwestern schools I can't remember which one and you know he has this joke that when physicists do math, they don't call it number science, right. And then this goes back to the good old data science craze days, and I think that if people would just hearken back to the scientific method and well-known issues in statistics, like variants, we would all be in a lot better shape as well. So, yes, I agree, it's about the fundamentals in many ways.
Speaker 3:Well, thank you so much for your time, patrick. This has been an awesome episode. Anyone you want to shout out, any references you want to give, we'll throw them in the show notes. But anyone you want to call out.
Speaker 2:Yeah, I'd highlight that NIST is starting a new sociotechnical AI evaluation called ARIA A-R-I-A, and you know I don't want to say too much about it. It's in early days but definitely getting ramped up, and they're certainly seeking participants in this real-world AI evaluation. That's not going to be a Kaggle leaderboard, it's going to be about real leaderboard. It's going to be about real world performance, and so you may be interested. If you're a vendor, you may be interested in participating in that, and if you're a consumer, you may be interested in watching the results of that. And then I'll say, a person that I'm drawing a lot of inspiration from these days is Ed Zitron. His podcast is Better Offline, and if you're a salty, cranky person about AI, like I am, then I suspect that you would really enjoy Mr Zitron's podcast. So check that out.
Speaker 4:Thank you so much, patrick. This was such a pleasure to have you on the show and you're welcome back whenever If there's any topic that you'd love to talk through. We'd really love to have you back.
Speaker 2:Sounds good, sounds good. Thanks for having me.
Speaker 1:Thanks and for our listeners. If you have any questions about this or any of our other episodes, please find us at wwwmonotarai slash podcasts. Until next time.