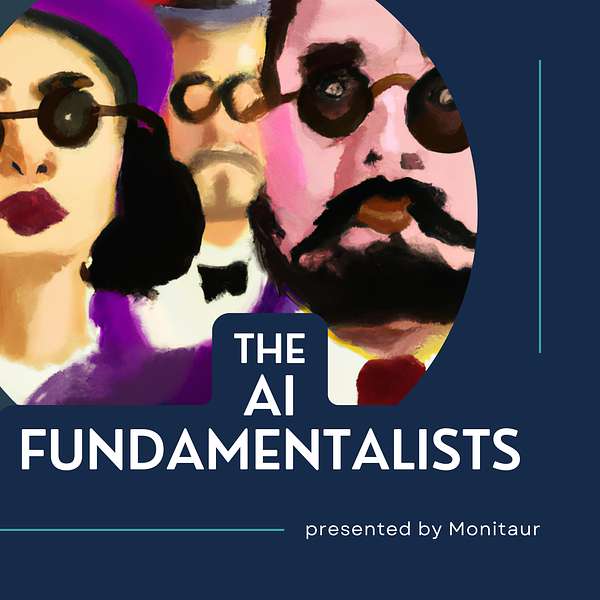
The AI Fundamentalists
A podcast about the fundamentals of safe and resilient modeling systems behind the AI that impacts our lives and our businesses.
The AI Fundamentalists
New paths in AI: Rethinking LLMs and model risk strategies
Are businesses ready for large language models as a path to AI? In this episode, the hosts reflect on the past year of what has changed and what hasn’t changed in the world of LLMs. Join us as we debunk the latest myths and emphasize the importance of robust risk management in AI integration. The good news is that many decisions about adoption have forced businesses to discuss their future and impact in the face of emerging technology. You won't want to miss this discussion.
- Intro and news: The veto of California's AI Safety Bill (00:00:03)
- Can state-specific AI regulations really protect consumers, or do they risk stifling innovation? (Gov. Newsome's response)
- Veto highlights the critical need for risk-based regulations that don't rely solely on the size and cost of language models
- Arguments to be made for a cohesive national framework that ensures consistent AI regulation across the United States
- Are businesses ready to embrace large language models, or are they underestimating the challenges? (00:08:35)
- The myth that acquiring a foundational model is a quick fix for productivity woes
- The essential role of robust risk management strategies, especially in sensitive sectors handling personal data
- Review of model cards, Open AI's system cards, and the importance of thorough testing, validation, and stricter regulations to prevent a false sense of security
- Transparency alone is not enough; objective assessments are crucial for genuine progress in AI integration
- From hallucinations in language models to ethical energy use, we tackle some of the most pressing problems in AI today (00:16:29)
- Reinforcement learning with annotators and the controversial use of other models for review
- Jan LeCun's energy systems and retrieval-augmented generation (RAG) offer intriguing alternatives that could reshape modeling approaches
- The ethics of advancing AI technologies, consider the parallels with past monumental achievements and the responsible allocation of resources (00:26:49)
- There is good news about developments and lessons learned from LLMs; but there is also a long way to go.
- Our original predictions in episode 2 for LLMs still reigns true: “Reasonable expectations of LLMs: Where truth matters and risk tolerance is low, LLMs will not be a good fit”
- With increased hype and awareness from LLMs came varying levels of interest in how all model types and their impacts are governed in a business.
What did you think? Let us know.
Do you have a question or a discussion topic for the AI Fundamentalists? Connect with them to comment on your favorite topics:
- LinkedIn - Episode summaries, shares of cited articles, and more.
- YouTube - Was it something that we said? Good. Share your favorite quotes.
- Visit our page - see past episodes and submit your feedback! It continues to inspire future episodes.
The AI Fundamentalists a podcast about the fundamentals of safe and resilient modeling systems behind the AI that impacts our lives and our businesses. Here are your hosts, andrew Clark and Sid Mungalik. Hey everyone, we're back and we've also had a chance to do a lot of learning, which is why we were revisiting LLMs. We realized it's been over a year since our last episode on the state of LLMs and what people were experiencing in that learning, but it seems like the more things change, the more things stay the same, and some of the things that we want to change are also staying the same. But first let's kick it off with the learning and look at the news. We are three days after the veto of the California AI Bill.
Speaker 2:Yeah, this was actually really surprising for me. The this veto came through Now California bill I mean, none of us are lawyers or experts when, because it hadn't been law yet, we just kind of cursory had read through the bill. So take that for what it is. We're not experts in what the text was specifically, but it was around the lines of very much like large language models, the cost of the models, like if it was a certain size, some of these regulations would go, which is kind of interesting. So basically it's targeting large foundational models or the users of large foundational models in a larger sense than like a small startup right, or a company just using a SaaS service. What was interesting?
Speaker 2:So it makes sense that Governor Newsom vetoed that because it doesn't really align with. It seemed very targeted against the big tech companies versus it being kind of like the risk of AI in general, and his veto letter it's like a three-page veto letter definitely discussed that hey, something should be more risk-based on what's the risk of the model. That's something we very much agree with and it's very analogous to what model risk management and things like that are, because sometimes the most impactful adversely to consumer models could be those smaller pricing algorithms, things like that that are AI but they may not even be foundational or using any sorts of language models, but they could just be traditional GLMs or basic decision trees or things like that that are technically AI that could have the higher impact adverse impact. So what was surprising is that California is not moving forward and, more specifically and we don't again know the procedural rules of California, uh, state legislator and things A lot of States allow the governor to uh give back edits.
Speaker 2:So it was a little bit surprising is that it was more like vetoing the whole thing and just saying, hey, I'm, I want to partner with the legislation, going forward versus giving back edits of like, if you're wanting to be risk-based, you could have come back with like hey guys, rework this and make it. If these are high risk models, you need to be doing the things that you describe and take it away from cost and the size of the model was what the anchor was and move it into a risk which was like. The points that Newsom brought up were the very much like anchoring on risk, which we agree with. I was just kind of surprised that again, don't know, maybe California, the governor, is not allowed to put things back. We'll have to look that up, but not recommending that just change the anchoring point versus just a full veto of the whole thing. So that kind of raised some questions on. You know, maybe Google and folks promised to help him with his reelection campaign or something.
Speaker 3:Yeah, definitely muddles the waters on his intentions a little bit, because we definitely agree that all models need to be regulated and guaranteed and governed. I don't think that means that we should dismiss some really good parts of the bill, which we're trying to set up protections for whistleblowers, trying to set up requirements for developers to perform risk assessments and then submit those to a government agency to then look over, and then using this argument, basically that it's like oh well, you know these types of legislations stifle innovation and prompt AI developers and you know that's going to make them leave the state. I don't totally buy that as like what's necessarily going to happen. We've talked about this before Innovation in this space and developers in and states aren't going to leave just because of regulations that require us to do it the right way. What we saw was basically a full-on veto and a letter saying like you know, this is the small reason, but also I have large interest in making sure that this is going freely in my state.
Speaker 1:What would your ideal bill look like?
Speaker 3:I think that I like a lot of the structure of the original bill. I think that, like it's a little bit troublesome that's also just going to be California specific. I would like to see something that like looks to build a more national model that the you know we have like a joint agreement on how this is going to work. I know there's a lot of pressure where, like, the strictest state becomes the only state policy and that becomes the national policy, and so it would have been nice to see this on a national floor level. It would have been nice to see, as Andrew was saying, including provisions for all models, not just simply basing it on cost. The cost is a nice shorthand, but I think it doesn't really assess the differential risk, because there are large models which do nothing and there are small models which make huge impacts on people's day-to-day lives.
Speaker 1:I agree with you on the. It needs to be at the national level. I am worried about a state-by-state like as much as I appreciated California really taking that step. I was really concerned. I'm really concerned with state by state. You know, unlike insurance, that regulates state by state Because there's physical space in each state that matters to some and not to others.
Speaker 1:I think when we get up to things like technology, you know there's no border between California and Nevada on how that models the risk of that model.
Speaker 1:You know there's no border between California and Nevada on how that model is the risk of that model. So I am concerned that it would go a little bit in the direction of, if we try to do state by state on AI regulation, it would go in the direction of the cannabis industry where you have like legalization state by state, but then say you know someone's flying, they take pot onto a plane and they get caught in federal airspace. There's now a federal fine and then they're fined for every state that they flew over. That is not where marijuana is not legal. So I bring that analogy up to say all we could be setting ourselves up for is double fines in areas that are just too muddy to regulate, that then become too muddy to regulate and just get bogged down in bureaucracy, and then we haven't done anything to prevent the risk yeah, I totally agree there and on this bigger point especially that legislation of the internet at the state level is basically a failed project.
Speaker 3:From the start. People have tried this. I think there has been some like content stuff where it's oh, ohio doesn't want certain content in the state, maybe a company will do it, maybe a company just won't do it. It's almost a failed problem because insurance, your car is driven in a state, but your internet is global. You're touching access points all over the world. When you access the internet, you're not just accessing like, hey, I want this website drawn for me from Idaho. That's not how the internet functionally works.
Speaker 1:Exactly From our point of view. We hope this means that they're going back to the drawing table and coming up with something, or it does inspire something at a much larger scale to make sure that we get regulation on the risks in place From the learning. We also see, as we mentioned at the top of the episode, llms revisiting what's been happening, a good segue from regulation and you know the whole try again.
Speaker 3:Yeah, I'm excited to catch up with the group here again. It's been about a year and a half. This is our second episode of this podcast ever was talking about LLMs, so we thought we'd take the opportunity today to talk a little bit about what has changed in the LM space and you know, like small spoiler, what hasn't changed in the LM space. Where have we gone and where have we stayed the same, when have our predictions held true and where do we see this going forward? So we'll start off with the first question, which is the question we opened with last time, which is can LMs be regulated, monitored or assured? And if so, what's that going to look like?
Speaker 2:I definitely think, as we thought this last time, and just the more that we've been experiencing this, we can double down and say, yes, they definitely can be governed and they can be monitored and assured. It's just a lot of work. And one of the siren song essentially, if you will, of large language models is this idea that businesses can buy a foundational model and it will solve a lot of problems and they can just plug it in and it's going to make their employees more productive. It's going to just make their companies better. They can build models off of it and it's just this large thing that they bought from a big company like Google and it's good to go. It's just this large thing that they bought from a big company like Google and it's good to go. That's not the case, and that's where that anchoring to risk versus it being the cost is really the key differentiator. On an anchor on this is because how is this model being used? If it's being used within helping you spell check emails and things like that but there's an end user actually reviewing that and then hitting okay, that sounds good. That sounds like Andrew writing an email and I misspelled this word and it cleaned it up Beautiful, that's great. You can just use it and enhance productivity that way.
Speaker 2:However, it gets very dangerous when you think, cool, I bought this thing, now let me put all of this PAI data from my customers into this model. There's IT concerns there that need to be addressed. That's not actually model specific, but take that aside. But then you're actually building models that are facing end users without a human in the loop, and they're automating decisions like let me try and do this for claims underwriting or let me try and make a pricing algorithm after this, or what have you. It gets very dangerous because those are now models except unlike traditional models that are very confined spaces and only work with certain data inputs and things like that, where you automatically have some constraints in place and still, after the uh in banking and things, you have OCC 2011-12 and and all these large regulations around how to evaluate and and do all these lines of defense and validate modeling systems that are these like dumb models that are very specific.
Speaker 2:Now you have these large models where you can kind of put anything in and get anything out, and you have all these hallucination concerns or like literally the latest nist publication concerns about people making biological weapons and stuff, asking a chat gpt how to do those things like these big open ended models. So now you need to put those same sort of guardrails to be comfortable using this in an automated space. But so you can definitely do that. But that's now taking away what that siren song that all the corporate executives love like let's automate this, let's just buy this thing and make it go away. That's not the case. You have to the controlling. You definitely can. You can make these auditable, governable, assurable, but it's almost more work at times. And is the cost benefit really there? Assurable, but it's almost more work at times. And is the cost benefit really there? And that's what a lot of the industry seems to be struggling with right now.
Speaker 3:Yeah, and we see these groups. You know that take on these large models and use them. And then you know what do they say? And the point is like is this model safe? Maybe they'll point to the model having a system card. Right, google left us with this idea of model cards, which is that you release these large foundational models, you give it like a little bit of a nutritional label and you say, hey, this is what roughly went into the model, here's roughly how it acts and here's like its report card in school. And then we say like, oh well, that just basically gives me clearance for all of my use cases. No, that's not how that's going to work. You need to validate and govern your specific use case that's built on top of the LLM, to make sure that it meets your compliance goals. And we would contend that the model report card that people are pointing to isn't even enough governance in the first place.
Speaker 2:That's a very key point because now model cards are 100% a step in the right direction, fully agree with that. But it is definitely not enough, and what's even telling is even OpenAI knows it's not enough because they did more than that in their latest system. They call it system card, not a model card, and I love that wording. Still, there's even not enough there. But the key part is I can put whatever I want on there and this is why you know FDA regulates foods and does spot checks. You can't just say USDA organic for fun, like we're at the place where a developer can say whatever they want on there and you can. As we've said, we always try and bring things back statistics here. Statists can say whatever you want them to say. Right, it's. You can make metrics that you can set a super low bar and pass it with flying colors and put that on as your in your nutritional label. But who's verified that? Those are actually the parts and and the key part of this risk-based approach is that objective assessment as well as how are you validating it? And looking outside the happy case, for instance, I can make a nutritional label for these model cards that say, hey, I just put in some really nice clean data into this LLM and it constantly gave me I gave it a list of US presidents and say, pick the third president in the list. Oh, and it got it right and I just did that a variation there. So I'm going to say the accuracy and retrieval score is really high.
Speaker 2:However, what happens if someone misspells things or someone puts something adversarial in there? Does it really go off the rails? Like you know, we have lots of examples of that happening and none of that the problems don't get addressed. So that's the model cards. I think it's a good trend, but it's now being said, oh, we're doing transparent, we're doing responsible. I don't agree with that. You're actually giving a false sense of security and that's where, like, going back to the regulations, like you're not really going to ever get that until, like, if California does something, that's every state's going to kind of have to follow it. To Sid's point from the like the internet regulation. But model cards give a false sense of security, I think. So they can honestly be more dangerous than not having anything. But that's just my opinion. But even OpenAI who, as listeners of the show know, we're not the biggest fans of their latest system card has describing how they red teamed and things like that, which is an amazing step in the right direction of adversarial testing a system.
Speaker 3:You know, maybe this is what legislation looks like, right? Maybe that ideal bill is. If you're going to build a system card, it needs to meet these goals, it has to have these compliances and it has to be standardized so that every group is expected to do the same things and we know what we're going to get. We were lucky that OpenAI did red teaming, but that wasn't required of them or guaranteed.
Speaker 2:Exactly and this is where, same as, like US, california and Virginia have adopted a variation of GDPR as an example that kind of set the global minimum standard and honestly for large tech companies building these models, euai Act, which really goes into effect in 26, 2026, is kind of honestly going to set the standard for Google and folks anyway. So if California literally white labeled and may change a couple of things from EUAI Act, just do that, because that's going to then just make the US have essentially have to follow those. And EUAI Act does the risk-based approach and requires it's not like very explicit, but it requires like the conformity assessments, independent evaluations and all those different components that help you lead down that good outcome evaluations and all those different components that help you lead down that good outcome Absolutely.
Speaker 3:And so, jumping off into our next topic we talked about last time. We talked about this was hallucinations, and looking through the old script, I saw that we even linked to the Wikipedia article for hallucination, because it's such a new idea. People weren't in consensus about what this was. There's no one in this AI space that doesn't know this term and doesn't have a personal definition. So I would go ahead and say I'm willing to make a new definition for hallucinations. I think we've kind of spent some time on it, we've kind of refined it and if I had to say it plainly, I would say that hallucinations are statements from an LLM that are unfounded based on its training data, and I think this really shows that we've kind of come back to seeing these as models and moved away from seeing them as magic. We really understand that what hallucination is is basically and I'll put this more academically later, but it's been called basically bullshitting, and the model is basically making up responses.
Speaker 2:Yeah, I think that's a great definition of it. And along your new definition said the latest NIST publication around Gen AI calls it confabulations, hallucinations or fabrications. So it's very much like that trend of the new definition you had is very much the industry is going towards that we're past. Even everybody understands hallucinations now. Now we're refining it even further. So that's a really good development to see over the last year and a half.
Speaker 3:And so the follow-up question is like okay, great, we finally understand hallucination, we know what it is, we have a great definition of it. Have we solved this problem? Not at all. Hallucinations are still rampant, they're still wild, they're still in the best models they have out there. Have they gotten a little better? They've gotten a little better, but they're still fundamentally there and they will still happen with some regularity when using these models.
Speaker 3:There are maybe two promising responses to this. The first approach which we this is actually a ladder approach we saw from OpenAI with these new thinking models or rationing models, which are basically we're doing the same reinforcement learning we were doing before, but now the annotators have to give explanations. This is better, a step in the right direction. There are some questions about the ethics of how they collected this data. This isn't really going to fix the problem. This is just going to give it better explaining abilities.
Speaker 3:And the other, and maybe more controversial, solution to this problem is, if you want to fix hallucinating, have another LLM read the output of that model and then correct it Basically LLM critique and you might say like, well, hold on, this is just like a rushing Nest, nestling all of the same problem, and to that I would say yes, yes, it absolutely is. Academic studies have shown that this LLM critique does improve the model. It does not make it perfect, it does not fix the problem, but it does at least lower the rate of hallucination. I think we're going to be seeing a lot more of the latter. I think we're going to see a lot of these LLM critique systems, because it kind of just lets us keep pushing the envelope and keeps us, lets us keep pushing on LLM, llm, llm without having to solve the problem.
Speaker 2:Jan LeCun has had some thoughts on this the head of research at Meta and I kind of agree which, like the real paradigm we have here is predicting the next word for a lot of these LLMs. Right, so now the Russian nesting dolls? Sure it works, it is a better approach to what we have, but it is a little concerning and then like the explainability and like LLMs explainable anyway, it just really goes out the window has said the same kind of thing as well, which, like, we really need to rethink this paradigm If we're really wanting to keep going down this path. Like probably explore more more fundamental model paradigms, like Jan LeCun is always into energy systems and things like that, just other approaches, because we're not really what people want an LLM to do, even LLMs, monitoring LLMs on top of LLMs we're still missing that actual. We don't have cognition or thinking or anything. It's just predicting the next word. So we're gonna max out performance with that thing unless we try a new paradigm like the whole real original idea of neural networks and trying to do things back in the day was you're trying to make a system like back to that?
Speaker 2:We had that consciousness conversation about a year ago in a podcast as well. Um it, we're nowhere near that, um, and we're definitely not anywhere near that with our current paradigm. So like I think it also needs to be like we can keep band-aiding for lack of a better word the current systems and they'll get better over time. But if what people really want these systems to do we fundamental research is still still has to happen, you're not going to be able to fully fix the hallucination problem. And with the current paradigms, maybe to a point, with a lot of effort to make it use more usable, versus, say, you know, 90% non-hallucination rate, maybe we can get a lot higher, but we're not going to get that a hundred percent under the current paradigms. And just like, if people are okay with that, that's fine, but that's. There's a lot of misinformation in the space of like how these systems are versus knowing they're really only ever going to.
Speaker 1:So that brings up the point about governance of these systems is that if you're going to do model on model, even in the case of like LLMs, critiquing LLMs, that just really elevates your case, for like you can't. Governance of a particular model is not enough.
Speaker 2:You really have to look top down into the system and have a full process for that you have to have the policies, the procedures, and then that's a great point you need to kind of you got to if you're governing the LLM with an LLM, you got to govern the LLM, that's governing the LLM. So it's like you just make your burden more and more and then at some point you got to step back and say, hey, is this really worth it? Or should I have built that smaller model in the first place? Or I'm kind of like a one. But should you just be using a knowledge graph if performance is really a high thing, using a knowledge database with semantic searching or something? If you really care about performance, you probably shouldn't be using an LLM. So it really just comes back to that type of scenario.
Speaker 1:And that's a great segue into knowledge graphs.
Speaker 3:Yeah, that's perfect. So yeah, basically, the next prediction that we made in that episode was that, coming from the standpoint of AI fundamentalists trying to do things the hard way, trying to do things the correct way it seems like a more correct approach to this would be knowledge graphs and databases as a solution to hallucinations. Right, because these are strictly grounded in realities. They're strictly grounded in documents that actually exist. And what did we see in the industry? We saw RAG retrieval, augmented generation. We see a little bit of this push to make these Gen AI models act like knowledge graphs and databases. Now, that does not make them the same. That does not make them immune to a lot of problems. But if your use case is strictly productivity tools like an AI assistant tool that's inside of your company that helps you hey, how does this code work? Hey, what's in this database that honestly might be enough. And this kind of reflects the idea that, like we don't want just the LM to run rampant, we want it to actually point to real materials.
Speaker 2:Exactly. And that's what's crazy is like RAG is such like a commonplace quote unquote thing now and at least in the space. And when we did our first LLM conversation, rags really weren't a thing yet, or I mean in academic circles, but they weren't like accepted of like companies talking about rags and using rags. It was very on the infancy at that point. So that's really interesting that people are trying to go that way.
Speaker 2:You still like a lot of the studies are still showing, you know, 90% accuracy on those. It's not a replacement for the other areas, but it's definitely a trend in the right direction versus the original idea of let's just use OpenAI's chat GPT for everything. Companies now are really like whoa, whoa, whoa. I should be using it at least on my internal documents and trying to get a little bit better from there. So that's definitely an improvement in the right direction, but again, it's still you're missing the forest or the trees a little bit, depending on what your goals are. That's where my general thesis is LLMs are great for productivity enhancing, but you cannot use them for these mission critical applications. You're best off using something else, because the LLMs monitoring LLMs and then governing all of that, and then and you're still going to have accuracy issues versus guys. Just build a model the hard way the first time and it's actually going to be less money, less time, less effort than trying to use an LLM to square peg round hole type thing.
Speaker 3:Yeah, absolutely, and I'll just give my subjective experience here. I've used a couple of these and I'm sure we all have access to Copilot, which is the free one that's available to us. It's right 80 to 90% of the time for getting work done. That's enormous. That's a lot of great extra work you can get done. That's shell scripts, that's sql queries, that's python code. It'll help you do all of that, but it's still only 90 accurate. You still need high quality talent inside of your organization to then use this knowledge and then know when it's wrong and to be able to read it and check it. So if your goal is to build an AI system to replace an engineer, this is not going to be it. This is going to help your existing engineers be better engineers or faster engineers, but you are not at the point where we're looking at replacing talent at all. This is basically an augmentation, but definitely nowhere near a replacement yet.
Speaker 1:Adding to this objective example, like one of I was in a conference and one of the things that the presenter brought up she was the topic was driverless car, like AI and like really, but like the physical space, like driverless cars. And you know her. Her first point was it's driverless, but the room, the big room, with people behind computer screens monitoring and watching and things like that really emphasize it, drove home the point for her on how the job will change With depending on how the technology is used. And you know that hit it home for me.
Speaker 2:I think that's great. I love that example and I think that's just what people I often get the impression. People think I'm like anti-LLM. I'm not. I think they're great. It's the how are they used and I just think the whole conversation as a whole industry, or just even at large, and even the whole country and world change what you're thinking LLMs are going to do. L and world change what you're thinking llms are going to do.
Speaker 2:Llms are great for productivity, productivity enhancement and we're getting into better paradigms for that. They're great for that. They will augment productivity. They'll make workers more productive. They might even change slightly some roles, uh, uh, and that's. That's a good thing. However, we need to stop thinking they're this panacea that are going to do things that are not built to do. They were never built to be replacing experts and that's where I think we got. We've kind of been chasing that siren song. That's incorrect use of them versus like self-driving cars to help be a little bit. You know, you're not not replacing the driver, but maybe letting you check your phone for a minute while you're still have your hands on the wheel.
Speaker 2:Like I don't know, tesla's trying to kind of throw the needle there. But enhancing productivity of a person versus trying to replace people?
Speaker 3:Yeah, absolutely. Let's hop over to our next topic, which is basically predictions. We didn't make Predictions that I wish we would have made, because I would have made a lot of money.
Speaker 3:We did not anticipate the scale at which tech companies would latch onto this and make it their main, or only, business priority. And with that came two things One, the massive escalation in production of GPUs and two, the unprecedented use of energy. You know, crypto was an enormous boom but, like the energy usage being talked about with trainees, lms is truly insane. We have meta on microsoft going on the records in earning calls talking about we're going to recommission or reactivate or build new nuclear power plants just to meet the energy needs of training the new llama. For, uh, I think microsoft has already committed to the uh, the since commissioned a three island nuclear plant, uh, and met us talking about building a new one.
Speaker 1:Yeah, this is that's unreal yeah, the three mile island news hit close to home, because I've lived up in pennsylvania for a long time and that was, you know, fascinating to see. But also remember them being shut down because you know there were better sources of energy and now all of a sudden we need energy so bad. Let's reactivate the one that we said was bad 20, 30, 40 years ago. So this is where ethics is also going to come into play, like when we talk about AI ethics. This is what's being talked about. Is that that energy better spent on a big tech company building more models for an unclear goal? Or is it better to ship that energy or use a better energy source to you know, fuel a third world country or fuel our country country? Look at what's happening now and at the time of this recording, we have half of the southeast without power and water.
Speaker 2:So when we talk about ethics and technology that ai has brought to light, this is exactly what's being discussed for sure and that's what's so wild is like these are like the people that would be lobbying to shut down the nuclear power plants are like, start them back up because we need to train an llm. It's. It's just mind-boggling of like guys, what is the objective here and what are we really gonna? So we're gonna get an llm to from 80 to 90 accurate to 91 accuracy and we're gonna be doing all this harm to do so. Like, come on, like also, how much?
Speaker 2:How many researchers could you be paying to figure out the new paradigm that doesn't require as much energy, versus just throwing like not normally do you just throw more something at a problem to make it, make it go away. Like this is just so different from normally how people have been and saying scientific advancement, this is like going backwards. So it's like, instead of trying like hey, let's rephrase this and try and find a better solution to the problem and do some fundamentals research, let's just throw more resources. It's just mind boggling how this is developing.
Speaker 3:Yeah, absolutely. It almost feels like a paperclip machine experiment If anyone's heard about that one where it's like you know, you have an AI system and it's told to build paperclips and it says, do whatever you want. And it's like, okay, well, I'll do anything, I'll start wars, I'll source aluminum from other planets, and it's like it's like let's do anything we can do to get this lms working. And it's like you have to remember that the transformer model block, it's an n squared operation. It's extremely expensive. There we've done some things to make it more efficient, but like, oh my god, we're just hammering this with everything we have and these nuclear power plants, they're not online yet. We're doing all this in unclean energy sources today and probably for the next few years, before we're ready to transition over to something else.
Speaker 1:Exactly, and I think at this point in time, the biggest question is innovation. For what is still the outstanding question on all of this? Even in the small increments that have been made to use AI as an emerging technology, there's still just that unanswered question on to what end.
Speaker 2:Well, let's also I've probably broken record people are tired of. But think about the Apollo program at NASA. Anybody's smartphone is so much more than computing power than what got the astronauts to the moon and back. Like the amount of computing power than what got the astronauts to the moon and back. Like the amount of computing power and things and the amount of resources. There is so much less than we're talking about here to land people on the moon. Like think how many small expert driven teams building expert models using glms, xg, boost whatever new model paradigm you come up with you could be doing versus restarting a nuclear power plant for who knows what reason for these models. Like it's just mind boggling of. Like let's invest in better tech leadership talent, better tech resources and like the amount of money you could be doing to upscale the workforce, be able to build target models that are 98% performance versus 80 to 90. It's ridiculous. I don't understand why these decisions are being made the way they're being made.
Speaker 1:For all that we just said on the to be continued, there's good news.
Speaker 3:That's right. So let's hit some of those good news points, which is basically like OK, you know, people were just like sitting on their hands for a year and a half, what actually happened in this field. What's better about LLMs that we didn't have before? Better about LMs that we didn't have before? I think that, just for people that are in this industry, I think that, very frankly, a lot of cool and fun projects have come around from this. I think there's a lot of jobs that were lost and simultaneously created, and some of these new positions they're just fun, right. It's just like, hey, you're going to do NLP problems and you can use this large model or you're going to solve things which weren't possible before, and I think that's been a lot of exciting and really invigorating for a lot of new talent and also people who have been in this field for a while that want to do something new. So, subjectively, there's been some fun to be had.
Speaker 3:We also have seen that these models have become less democratized than they were before. They're not a hugging face anymore. There's no more GPT-2 type access, but we still have access to a lot of these models. There are the Laman models, which you don't get the weights anymore. But you can still use really, really large models that they're not going to fit on your desktop. They're not going to fit on like a small company server. You're going to have to spin up a small little farm to use them and API pricing is still pretty reasonable. It's not yet at a point where companies are like, oh well, my little Gen AI startup isn't possible, you can still do all this. Is this forever? Maybe not, but for now these models are still relatively usable.
Speaker 3:We've also seen really big improvements in multimodal modeling. Stuff that wasn't possible before is now relatively easy. Right, the same LM models we had before that were just doing image or just doing video. We've seen opportunities for us to merge these models and have unified models that can talk to you and write messages to you and send you images and understand video. That's really exciting. That's really interesting for people that work in human-computer interactions, helping people with disabilities things that weren't possible before. These are really amazing breakthroughs.
Speaker 3:We've seen good models get smaller Models which far exceed GPT-2 now fit on your phone. That's awesome. Yeah, and as mentioned before, if anyone was using Lama 2, Lama 3.1 and 3.2, they're absolutely enormous. These are like many, many millions of parameters and they've given us three different small, medium, large, One that's like a laptop size, one that's like a desktop size and one that's like you need a server farm and you can use that model and run it and fine tune it to your use case. And now you don't have the same power as an open AI, but you can do a lot of what they can do at home and without having to give your data to anyone else. So I think there's plenty to be excited about and happy about in this space. It's not all doom and gloom. I think a lot of the fundamental problems aren't there and these models have gotten at least more interesting and better.
Speaker 1:Yeah, and there's no shortage of topics to be discussed. Coming forward because we're going to be seeing, like we mentioned earlier on, the model-on-model case discussed. Coming forward because we're going to be seeing, like we mentioned earlier on, the model on model case, um and agent agentic modeling. Um is going to be a key experiment for a lot of businesses this year as they try to figure this out.
Speaker 3:Yeah, absolutely this was. There's been some really great and exciting cool agent work, uh, which we'll get into later, but like models worked with models to solve problems, uh, and some really cool stuff has happened there too.
Speaker 2:To really do agent-based modeling. That's a fundamentalist thing. You can't just say, hey, llm, make me a big, large agent-based modeling. It's agent-based modeling is complex, so it's definitely a very fun evolving space. It's really been taking off like the past 10, 15 years and just getting more so as well, and computing powers really helped it because those are computationally challenging as well. But that's going to be a very interesting difference and, like the intersection between elements will be interesting. But there's a lot of research. It's one of the more active areas of modeling right now, even in the traditional modeling space.
Speaker 1:You know, in the spirit of the more things change, the more some things have stayed the same, like what's our conclusion from the last episode.
Speaker 3:I'll go ahead and read you the same conclusion verbatim that I had written down for the last time I did this and we'll see how I feel about it. Reasonable expectations of LLMs. Where truth matters and risk tolerance is low, LLMs will not be a good fit and I would say I still stand by that. I would say that's basically the same fundamentals we were dealing with before, same issues we've been dealing with before and while they've gotten a little bit better, I think that still holds. You should not expect an LM can do a high risk operation without a human in the loop or without guidance without a human in a loop or without guidance.
Speaker 2:I think that's genius. Yeah, our main conclusion lots happening, nuclear power plants opening up, lots of money being spent. We're fundamentally the same place. Yeah, we got better memes than we had a year and a half ago. Memes have gotten really good with these systems, memes have really upgraded. But like, is that really helping society in general? Is that really where we want to be going? Probably not. Are these doing what people? Have we gotten any closer to the panacea that people are wanting these models to do? No, but they're smaller, they're faster, they're better.
Speaker 1:But conclusion is the same don't use them in mission critical applications and, if anything, we've had the benefit of almost a year and a half since that episode to see this actually playing out in work that we do with models and the model builders that we work with.
Speaker 2:Exactly. And guess what? Ai governance is the same. It's just harder with LLMs, but it's the same process. It's nothing new. There's nothing like. A lot of times we get like, well, it's different, it's not really different, it's just more work because you are having a broader surface area. But it's not, it's. You still define the use case, independent review, risk assess, make sure you have correct monitoring place. Did you validate it for the use case? Like those same blocking and tackling. It's the same. It's the same. Occ wrote in 2010 it's the same stuff over and over and over and over again. It's just not a popular answer, but it's just hard work. Modeling is hard and so far, despite the nuclear power plants opening, we have not had a way to make modeling easier for mission-critical applications.
Speaker 1:I would add that that conclusion stays the same and what it's done for better or worse is, for most people, foundational models and generative AI might be their first business foray into any AI. We've traditionally worked with companies who have had modeling paradigms and modeling systems from other modeling types, but when you get to this being net new and people learning over the past year, what it's done is it's made. You know, it's just brought to light. Now you have the board looking in on companies and wondering, like, what is our AI plan and what is the sustainability of this? How does this, you know, keep us relevant, keep us brand very loose, you know, very loose generalization. How does this keep us relevant into new markets and everything? So I think if anything has grown from that our original conclusion it's just the heightened visibility and everybody wanting to understand what this is or isn't going to do for their future.
Speaker 2:That's an amazing point, susan Fully agree, like I think that is. We should have had that as one of the net positives. Even the fact that we talked open with California regulation maybe maybe not happening. Eu AI, all these things the fact that Gen AI and LLMs are around the last year and a half has so increased the people concerned about model governance and AI governance in general, and that is capturing models that people may not have realized were AI previously. So I think, yes, that is a major benefit of this conversation. I love that point and, yes, it's raising the profile of, hey, we need to make all of our models responsible, not even not just if it's in banking people have been doing that but outside of banking, we need to address all of these concerns and make sure we're not doing adverse impacts against individuals. So that's an amazing development.
Speaker 1:I think that I think we've covered it. I'm actually I was actually looking forward to this episode because, like just doing the research for it on, like you know you're so busy, you know we're so busy, and we go back and take a look at what's happened. This was fun to go through and on that, on that point about the complexity for our listeners it sounds trite to say, but we're here for you, we're here to help you grow into that next level of complexity with really helping models differentiate your business. If you have any questions, please leave comments in our chat, on our posts on LinkedIn and on YouTube. Until next time.