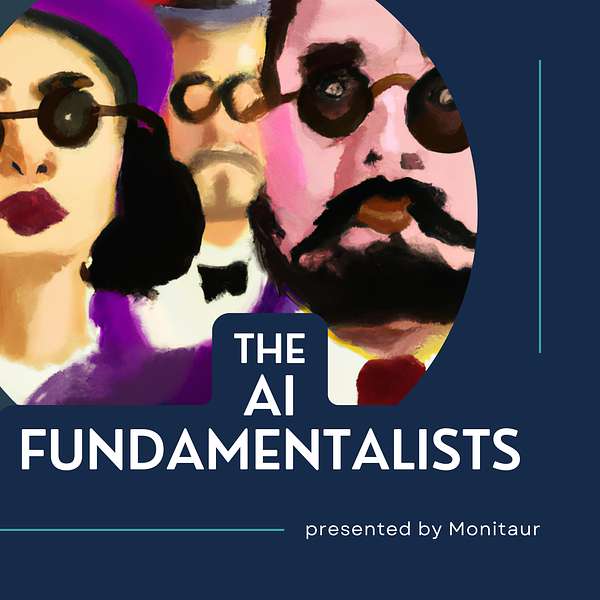
The AI Fundamentalists
A podcast about the fundamentals of safe and resilient modeling systems behind the AI that impacts our lives and our businesses.
The AI Fundamentalists
Contextual integrity and differential privacy: Theory vs. application with Sebastian Benthall
What if privacy could be as dynamic and socially aware as the communities it aims to protect? Sebastian Benthall, a senior research fellow from NYU’s Information Law Institute, shows us how privacy is complex. He uses Helen Nissenbaum’s work with contextual integrity and concepts in differential privacy to explain the complexity of privacy. Our talk explains how privacy is not just about protecting data but also about following social rules in different situations, from healthcare to education. These rules can change privacy regulations in big ways.
Show notes
Intro: Sebastian Benthall (0:03)
- Research: Designing Fiduciary Artificial Intelligence (Benthall, Shekman)
- Integrating Differential Privacy and Contextual Integrity (Benthall, Cummings)
Exploring differential privacy and contextual integrity (1:05)
- Discussion about the origins of each subject
- How are differential privacy and contextual integrity used to enforce each other?
Accepted context or legitimate context? (9:33)
- Does context develop from what society accepts over time?
- Approaches to determine situational context and legitimacy
Next steps in contextual integrity (13:35)
- Is privacy as we know it ending?
- Areas where integrated differential privacy and contextual integrity can help (Cummings)
Interpretations of differential privacy (14:30)
- Not a silver bullet
- New questions posed from NIST about its application
Privacy determined by social norms (20:25)
- Game theory and its potential for understanding social norms
Agents and governance: what will ultimately decide privacy? (25:27)
- Voluntary disclosures and the biases it can present towards groups that are least concerned with privacy
- Avoiding self-fulfilling prophecy from data and context
What did you think? Let us know.
Do you have a question or a discussion topic for the AI Fundamentalists? Connect with them to comment on your favorite topics:
- LinkedIn - Episode summaries, shares of cited articles, and more.
- YouTube - Was it something that we said? Good. Share your favorite quotes.
- Visit our page - see past episodes and submit your feedback! It continues to inspire future episodes.
The AI Fundamentalists a podcast about the fundamentals of safe and resilient modeling systems behind the AI that impacts our lives and our businesses. Here are your hosts, andrew Clark and Sid Mongolek. Hello everybody, welcome to this episode of the AI Fundamentalists. Today, we're covering topics in contextual integrity and differential privacy. To do that, we have a special guest. Sebastian Benthal, or Seb as we all know him, is a senior research fellow at the Information Law Institute from NYU School of Law and a research scientist at the International Computer Science Institute. He is also an external faculty member in NYU's agent-based modeling lab. Seb, welcome to the show.
Speaker 3:Glad to be here and we're super excited to have you on and talk a little bit about the work you've been doing. I think, specifically, we're really interested in your recent work. This is the work that you published in 2024, so just this year called Contextual. You know, Working with Contextual Integrity and Differential Privacy. The nice title is Integrating Differential Privacy and Contextual Integrity, and we really want to dig into this article. We saw that it was published in CS Law with Rachel Cummings from Columbia and just breaking it down piece by piece, walk us through a little bit of what is contextual integrity before we get into the other pieces and how they come in together.
Speaker 2:Sure. So contextual integrity is a way of thinking about privacy and really data protection more generally, especially as technology changes, and it evolved out of law and sociology and philosophy. It's really the creation of someone named Helen Nissenbaum, who's a professor now at Cornell Tech. One way of thinking about it is as a philosophy behind sectoral privacy laws in the US. So in the United States we've got like HIPAA governing health care, we've got GLBA governing finance. We've got a number of different laws that cover specific sectors of society, and the theory of contextual integrity says every context in society has a purpose and particular norms and when information is flowing in accordance to those norms, it's appropriate. So it's an idea of privacy as appropriate information flow. But imagine society as having many different contexts or sectors in which that standard is applied.
Speaker 3:Okay, that's really, you know, that's good and that's in line with what we were reading. So I want you to walk me a little bit through. What does it mean for information to flow appropriately in a context, right, maybe almost like a definition of what does appropriate mean and maybe like what is the context in which this information is flowing in?
Speaker 2:Those are the great questions. So, contextual integrity it draws on various forms of social theory and political philosophy, including Michael Walzer's spheres of justice and something like Pierre Bourdieu's theory of social fields. So a context is a socially understood field of activity. So being within the healthcare system, right, is a context. The family is another context. Right is a context. The family is another context. And context has within it roles defined.
Speaker 2:You know, in one case it might be doctors and patients, In another it might be parents and children, and the kind of social rules that apply in these different fields are different and are sort of keyed to the roles that are defined in those contexts. What is appropriate is a million-dollar question. To some extent it's what people already expect. But there's a question also of what is legitimate in terms of individual needs and also the goals of the context. So we expect, for example, in education, for there to be certain rules about, say, how students' grades are kept confidential or reported, and they should be in service to the goals of the education system actually educating students and providing credentials.
Speaker 3:I think that's really interesting and I think this kind of gets this underlying point that what makes up the appropriateness of a context or the appropriateness of information flowing in that context is defined by social norms which aren't constructed by individuals. There's some shared agreement that this context needs to operate in a certain way, based on expectations about privacy expectation and what's is and isn't allowed to be made public to other members in that context. So and I think you started to touch on this a little bit with the grades but how do we use this idea of contextual integrity in the real world and and where do we see it being applied? Either implicitly by people and then maybe more explicitly in design systems.
Speaker 2:So the original proposed use of contextual integrity was as a kind of design heuristic for reacting to changes in information flow that result from new technology. So, for example, remember smartphone-based contact tracing in the COVID-19 pandemic. There were a lot of questions about how that data should be handled, and one reason why that data was sensitive is because it introduced Bluetooth-based proximity data, a kind of new, kind of data that hadn't been normed around before, and people had to say, well, does this pose a privacy risk? What should the norms be around it? And there was a lot of disagreement about this, but in many places that are privacy conscious Europe especially they sort of settled on saying, well, we should collect this data in a way that is encrypted so that personal identification is hard to do, and we're going to use it essentially in service of the public health goals of the system, without allowing a lot of data to leak to say, the smartphone vendors for other sorts of purposes. So the connectional integrity is somewhat aligned with sort of GDPR styles of purpose limitations and other kinds of data minimization rules.
Speaker 2:Another interesting study that came out of MITRE this is Carl Bloom and Josiah Emery. They have a 2022 paper about privacy expectations for human autonomous vehicle interactions. So if you have autonomous vehicles out on the streets now, you've got new sensors collecting data. There's a question about how those should be regulated, and one use of contextual integrity that they employed was to use it and the way it sort of schematizes expectations, to structure surveys, to figure out what people's expectations of privacy were about that, even before these vehicles hit the streets, and there's ways that interacts with consumer protection law and the ftc to say, um, oh well, if people actually have these expectations and maybe the the regulation should work to reinforce those expectations- and so I guess, when we're talking about regulations built around contextual integrity, I think that there's really strong alignment between you know what we expect these systems to do and how we want the regulation to look.
Speaker 3:Does that bear out in regulators accepting CI as a concept or them talking about it directly?
Speaker 2:During the Obama administration there was some acknowledgement from the White House of contextual integrity and the importance of context sensitivity and privacy.
Speaker 2:Sadly for the contextual integrity research community it hasn't had a lot of uptake directly and often CI sees itself as opposed to the dominant view of privacy which in consumer protection, is notice and consent. So according to current law in the US and analogous laws elsewhere, if you're not in a particularly regulated sector, you can get away with basically anything as long as you write it into your terms of service or your privacy policy. And we know that there just are not enough days in the year literally for people to read all the privacy policies that they sign off on. And yet there's a legal fiction that people are engaging in, contracting and somehow we have an economy that depends on this legal fiction. The contextual integrity theorists argue that this sort of notice and consent is an end-run around privacy. They say there's no way for people to understand the complexity of what's going on with their information, with their information. We need stronger rules that don't depend on this fantasy of consent to a really complicated contrast.
Speaker 1:But that would require a change. I want to go back to. In everything that you just said in contextual integrity, there's a tail wagging the dog scenario for each of these, because are you regulating to the um accepted context, what people are just starting to accept this context, or is there? Is there a need for standard, like it doesn't matter what's starting to be accepted, we need to shift back. It's almost like an ethical conundrum. Any thoughts on that?
Speaker 2:I think you're hitting some really deep points that are issues that the CI community is currently wrestling with. It's a theory that's 20 years old and has been reacting to changes over time, as big data wasn't a big thing when the theory first came out. New privacy enhancing technologies are more recent inventions than this theory. So one thing the contextual integrity community is doing now is grouping together and coming up with new standards for what CI ought to mean, and part of that means addressing these kinds of questions. So the original theory kind of assumed that there were long-standing traditions in various contexts. That would be the friction, the kind of social normative friction against various forms of change and erosions of privacy. As things are so turned on their head because every five years we have the introduction of a new system that seems to change people's expectations. We clearly need a more robust way of doing the ethical reasoning about this and a clear way of delineating where contexts begin and end and what happens if they overlap.
Speaker 2:There are many interesting examples here. There's a professor, Dara Hoffman, who does work on Indian territories, Native American territories within the US which have their own tribal customs that are different from, say, university library customs, and there's questions about how to deal with sensitive tribal records that might be in a university library but they want to reclaim them for the Native American reservation. So how do you deal with that kind of conflict of norms over the same kinds of records? My own take is that there's a lot of very interesting kind of complex systems and agent-based modeling work to be done to sort of draw out these dynamics of norm formation over time. And so my personal interest is in doing that kind of scientific work to really nail down, with computational sociology and economics where, how these norms might form and what legitimacy might mean economics, where how these norms might form and what legitimacy might mean.
Speaker 3:That's super interesting, I mean. I think this is almost adjacent to some of the work that we do over in the lab that I work with, where we computationally determine what norms exist out there in the universe, right, we look at social media environments and we see what people are independently saying unprompted, and extracting these social norms from people's latent speech. And so, as you look out on, you know a world where privacy is, you know, eroding and it's not. We're not really always aware of what is and isn't available to us. What are some of the things that are coming out of the CI world that are that next step, that are going to help us build past our old assumptions and get ready for this new world?
Speaker 2:Well, you brought up this work with Rachel Cummings about integrating differential privacy and contextual integrity, which I think has a lot of promise. I suppose I'm biased. The key issue there is that computer scientists, information theorists, privacy researchers understand what it means to have kind of a lossy channel of information. Contextual integrity doesn't have native to it a model of a partial flow of information and to some extent that reflects the fact that society as a whole doesn't have a very good sense of what it means to have a differentially private information flow. So there's a lot of interesting research in usable privacy and usable security.
Speaker 2:Now to say, is it possible to communicate kind of the details of these more nuanced privacy-intensing technologies that allow for data analytics to happen and privacy to be preserved?
Speaker 2:Is there a way for that to really become normative? I mean, the US Census has adopted it, so it is now legally the case, but that was a very controversial move. But even setting aside the kind of sociological question, there's also a computational question of how to tune the parameters of these complex systems to achieve the legitimate social goals of privacy, assuming that privacy does involve this kind of balancing act of interests and purposes that contextual integrity does, and for that I've been working with computational game theory frameworks to model out scenarios and try to figure out how to turn those tuning problems into an optimization problem that's well-defined. Now that's still kind of mid-stage work, but I think it's a promising direction direction and that if we can start really kind of modeling what's at stake with privacy and data use in a robust but comprehensive and nuanced way, we could make a lot of progress in designing systems to be not just compliant but really good.
Speaker 4:Yeah, that specifically is what I was really excited about when I saw your work on contextual integrity. Is that one of the big issues with differential privacy is it's a really cool technology that works well, but there's no like the fact that even NIST just came out with. You probably read the new NIST standards on differential privacy and like the key punchlines about well, how do you tune epsilon? Because you could make like the parameters. You can make it either very, very restrictive and it very much is secure for the individual, or you can be using differential privacy and it really doesn't do much of anything to help with privacy and it's just like, well, you could have even this technical spec that you would be differential using those methodologies. Well, you could have even this technical spec that you would be differential using those methodologies and then even the result is so broad you can still be compliant with that standard. So it's definitely to me it seemed like there was something missing on like there's no theoretical underpinning of how to actually do differential privacy.
Speaker 4:Well, and that's what I think, that contextual integrity in the paper you did with Rachel Cummings really like sets the stage of like, hey, this could be a way. Yeah, we gotta figure out the norms and all the game. Theoretic approaches, I think, is a great way that you're walking into what those are. We have to figure out what's the norms that people wanna have, but it's like the methodological framing for how you could effectively actually determine what those parameters are. So it's useful, because it's weird, we came up with a tooling for differential privacy before we figured out how to use it and now you get an artificial sense of privacy if you don't tune it properly.
Speaker 3:And I think, before we dig into the answer to that question, I guess I would really love to hear you put differential privacy in the context of intentional integrity and then we can talk a little bit more how they work together, because we did the nice little definition of contextual integrity. So let's talk a little bit about what is differential privacy and how those two pieces slot in together.
Speaker 2:Sure. So differential privacy is a definition of privacy, essentially on the database and on queries on that database and on queries on that database, and it depends on the condition that any particular person's entry in that database affects the outcome of the query with a bounded probability, and that probability bound is a threshold that is characterized by a particular parameter, epsilon, and as. Andrew was saying if that boundary is zero, then you essentially get no information about the person passing through to the end of the query at which point the differential privacy theorist would say that gives you maximum privacy and minimum utility.
Speaker 2:Alternatively, you could just set that threshold to infinity and it's still technically differentially private, but you're letting all the information through. So Cynthia Dwork and others that invented differential privacy did it because they wanted a context-free definition of privacy that would be secure against any kind of inferential attack based on any kind of inferential attack based on any kind of side information. And they've worked out the math of that. But people have in practice had these astronomical values like the bound is E to the power of epsilon, and if epsilon is 32, that's an incredibly high number. So one thing we're doing in this work that's still ongoing with Rachel Cummings is we're putting, we're trying to put differential privacy literally in context, and that means, among other things, trying to move past the privacy utility trade-off at the level of the mechanism and start looking at what do the social actors in that context want, what is utility to them and what is privacy to them? So that means that we can project from this narrow privacy utility trade-off mechanism to an actual trade-off between socially embedded utility values among a number of agents. This leads to a lot of well-known problems like social choice problems how do you balance the interests of a bunch of different people but it is more, in a sense, honest to the problem that's being addressed. And if you're able to make those certain assumptions about well, which actors are you really privileging or not, or are you weighting their outcomes? Or using some kind of multi-objective optimization, you can do things like tune the amount of noise that the mechanism produces. So another way that we're departing a bit from traditional differential privacy is, rather than focusing on the epsilon parameter or the bound, finding it's easier to just tune the amount of noise in the mechanism itself, which is closer to what an actual engineer would do anyway if implementing a system. We don't know yet what the reviewers will say about this approach, but I'm quite excited about it.
Speaker 3:I think this is really exciting and I think it really clearly tells the story of how we see differential privacy as a tool for basically enabling a continuous spectrum of privacy controls. And then combine that with the contextual integrity which includes that understanding of how much control do we need in a specific scenario gives us this blended system where we are reporting the amount of privacy that's required for the specific context that's being deployed in, and that seems like a really strong pairing.
Speaker 2:Thank you. I will say that it does bring up a lot of other methodological challenges. Now, once the genie is out of the bottle for saying okay, we're going to have the tuning of this depend on a model of not just the information flows but also people's privacy preferences Also, you know, a sense of sort of social payoff, also a sort of balancing act between different actors. There become a lot of free parameters which would take a lot of work to do to do well, and to some extent, one concern I have with the method, or limitation of it so far, is that collecting that information about the privacy preferences of the data subjects might be as compromising in some ways as collecting the data that they have these preferences about. But that is a hunch at this point and I state it just because I think it is a legitimate limitation of the method.
Speaker 4:I think this is great. I really love the new way of approaching it of not just focusing on the epsilon and the bounty. I'm very excited to see the new research you're doing on the noise component. That definitely seems very interesting. Yeah, I agree with the game theoretic underpinning that's going to be interesting and then trying to infer the utility of subjects without giving up too much privacy I are. I really love these all fresh perspectives and it's kind of seemed like the differential privacy has kind of hit kind of a wall on some of the research. I'm loving that you're bringing in other perspectives to try and find a way to get past that and make it actually because it's useful for like the US census and things where they control the number and say what it is. But it kind of gets into this position where companies can say we're doing something like differential privacy but then they're still using the same amount of personal information, but now they get like a hall pass that they're doing the thing that the US census is doing but nobody discloses the epsilon.
Speaker 2:Yeah, yeah, thanks. I mean, privacy washing in that sense is definitely something we're kind of on guard against. And I should say that there's the field of differential privacy. Research is very, very deep and rich and there are likely other people working on other angles on this problem that I'm not aware of, but we might be the ones most seriously bringing contextual integrity to bear on it.
Speaker 3:And then just looking forward a little bit, and maybe this is just in the realm of hypothesis. You know you probably haven't completed these experiments yet, but as we think about agents operating in this space, you know, voting for the level of privacy they want in some anonymized or unanonymized way, do you foresee a world where the ultimate decision is made by the person who's the most privacy concerned by? You know, basically a democratic vote or being skewed by some governing body?
Speaker 2:I'm a bit of a realist. So at the end of the day, I think a lot depends on who's in power. At the end of the day, I think a lot depends on who's in power and it's very hard to even if presenting an ideal, it's hard to bring something like that about without there being some really good faith actors trying to do it. But I wonder if some of what you've asked is a bit of a false dichotomy, because it might be possible to have individualized noise thresholds based on preferences. So it's not, like you know, some data subjects need to, you know, necessarily have to sacrifice their preferences or desires to others if we can sensibly do those trade-offs.
Speaker 2:One one thing we're finding as we're running these simulations is that indeed, if we, if we have sort of disclosures, be voluntary in the simulation, then we wind up with data that's biased in favor of those who are the least privacy conscious. But with a fully specified model the analysts can de-bias that assessment and take that into account or sort of oversample the more privacy conscious people so that allows them to get more out of the even noisy data that they have. So I'm optimistic about those kinds of issues. Example in the recent presidential election we found a lot of polling error again and arguably a lot of that polling error happened because of sampling issues biased sampling of the polls. So pollsters have been dealing with this kind of issue for some time. It's a good question whether some kind of privacy enhancing technology could improve that in the future.
Speaker 4:Yeah, is it self-fulfilling prophecy if samplers going to get or pollsters going to get people that they want to do it, or is it the people not wanting to reveal their intentions? That's very interesting and pretty much anything that we can do to help with polling. I think that that's definitely a good application and I think I love those thoughts. I think this could be something game-changing for the polling industry. I love all these new, these fresh perspectives again, like really bringing economic theory and agent-based modeling and game theory games and finding out people's hidden preferences and utilities and really bringing that all the economics to bear on this historically computer science program. I think that is great.
Speaker 4:And trying to find that methodological underpinning for that's going to be the longer conversation, obviously, but finding a way to better understand the context, right. So I love that you've just even that initial paper of setting the like hey, here's a different way to think about it and really just get it out of the computer science land, because it kind of seems like computer science land has pushed the concept as far as it could. For now, anyway, the gap is not. Of course we can go farther. The gap is not computational now. Uh, with regards to like the actual mechanism of making it private or not.
Speaker 2:It's like how to apply it is the biggest gap yeah, but ultimately all these computational systems are socio, sociotechnical systems when they really exist and that social complexity is something that the computer scientists to some extent draw a line around, what they do not all of them, but often based on what it is that they can solve without that social scientific knowledge. I think that many scholars of sort of socio-technical systems unfortunately wind up doing a lot of great anthropological and human-centered work but then don't take the step of rendering that computationally in a way that really brings it back to the system design. And what I really hope to do with my work moving forward is build out that computational apparatus for modeling the sociotechnical systems so that we can be really rigorous about designing those systems moving forward.
Speaker 1:Any final thoughts, either something that you're working on in the immediate future or something that you'd like to see from the academic community that you're working with in the short term.
Speaker 2:Good tooling. Good tooling for modeling socio-technical systems and doing some of the hard computational work of settling the strategic and kind of embedded. All agents within these systems are optimizing or rational, but it is equally naive to assume that they're acting in a sort of rule-based way that doesn't involve adaptation and dealing with those kinds of problems of optimization and statistical inference. There's a lot of work, prior work on that from the computational economics communities, but we don't yet have something that is as seamless or turnkey a way of doing that as we expect from more kind of robust and mature computational domains. So I'd love to see that.
Speaker 4:Just really enjoy this conversation. Always learn so much talking to you and very excited to hopefully have you back on the podcast in the future. Talk some of your great work you're doing on causal modeling and also just digging in more on this. What you just even closed with the agent-based and some of the gaps you see in some of the tooling. And yeah, definitely agree with you here on the tooling gaps, but it is definitely as a technologist trying to build software. I also understand why there's gaps in this tooling. It's a very, very, very complex problem and I know some of the other projects you're working on where you're trying to help mitigate this gap as well. So just really appreciate you coming on and I think you're always looking at problems in new ways and just really really enjoy following your research.
Speaker 2:Thank you so much and thanks for this opportunity. It's been great talking with you all.
Speaker 1:Yeah, it was a pleasure. Thank you, Seb, and for our listeners. If you have any questions about today's topic or any of the other topics in our episode catalog, please drop us a note or leave us a comment. Until next time.