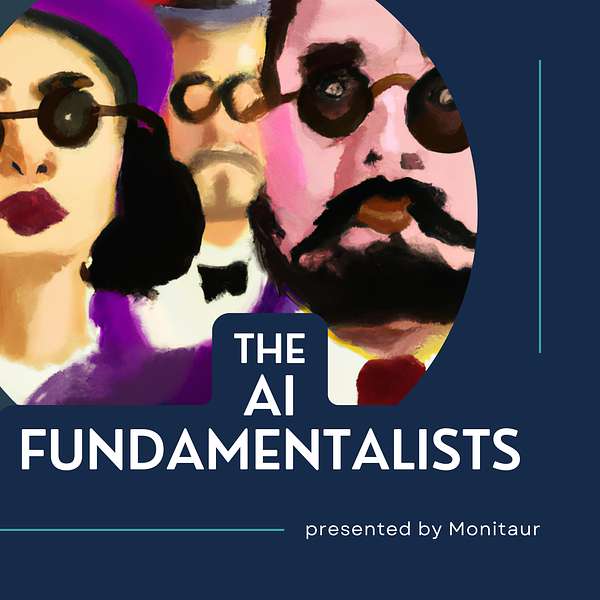
The AI Fundamentalists
A podcast about the fundamentals of safe and resilient modeling systems behind the AI that impacts our lives and our businesses.
The AI Fundamentalists
Agentic AI: Here we go again
Agentic AI is the latest foray into big-bet promises for businesses and society at large. While promising autonomy and efficiency, AI agents raise fundamental questions about their accuracy, governance, and the potential pitfalls of over-reliance on automation.
Does this story sound vaguely familiar? Hold that thought. This discussion about the over-under of certain promises is for you.
Show Notes
The economics of LLMs and DeepSeek R1 (00:00:03)
- Reviewing recent developments in AI technologies and their implications
- Discussing the impact of DeepSeek’s R1 model on the AI landscape, NVIDIA
The origins of agentic AI (00:07:12)
- Status quo of AI models to date: Is big tech backing away from promise of generative AI?
- Agentic AI designed to perceive, reason, act, and learn
Governance and agentic AI (00:13:12)
- Examining the tension between cost efficiency and performance risks [LangChain State of AI Agents Report]
- Highlighting governance concerns related to AI agents
Issues with agentic AI implementation (00:21:01)
- Considering the limitations of AI agents and their adoption in the workplace
- Analyzing real-world experiments with AI agent technologies, like Devin
What's next for complex and agentic AI systems (00:29:27)
- Offering insights on the cautious integration of these systems in business practices
- Encouraging a thoughtful approach to leveraging AI capabilities for measurable outcomes
What did you think? Let us know.
Do you have a question or a discussion topic for the AI Fundamentalists? Connect with them to comment on your favorite topics:
- LinkedIn - Episode summaries, shares of cited articles, and more.
- YouTube - Was it something that we said? Good. Share your favorite quotes.
- Visit our page - see past episodes and submit your feedback! It continues to inspire future episodes.
The AI Fundamentalists a podcast about the fundamentals of safe and resilient modeling systems behind the AI that impacts our lives and our businesses. Here are your hosts, andrew Clark and Sid Mungalik. Welcome to today's episode of the AI Fundamentalists, and this is a special episode for us because we are live together for the first time ever recording one of these episodes. That's almost been two years.
Speaker 2:Yeah, this is a big exciting moment. It's nice to not have the headphones on and just have a normal conversation.
Speaker 1:Yeah, and we also have the perfect timing of being in front of some very big AI news this week.
Speaker 2:but just as we had planned to record this episode about AI agents, yeah, so I'll tee us up a little bit and then let's hop into some news, because I think that everyone kind of knows what we're going to be talking about Today. We'll be talking about AI agents. I want to talk about an AI agent that's a system that uses a machine learning model like an LLM, but traditionally using older systems, to decide the control flow of an application or to make autonomous decisions about a system. This is rather than the traditional ways that we do, like predictive modeling or generate images or language. We want, basically, the agent to do everything for us, right, to basically operate like a person would.
Speaker 3:We'll also be releasing an upcoming research brief for our clients on NGINTIQ AI. So I'm excited for that to be coming on, our first research brief we'll be doing, trying to aim for one quarterly this year. So we will really dive deep into like the background of how did it come about, what are some of the underlying methodologies and kind of where are some of the inspirations, and get into a little bit to the more of the math and things that we traditionally do with some of our podcasts and blog posts. So very excited to be releasing that shortly.
Speaker 2:And so with that let's get into the news a little bit. I think that everyone's been hearing about DeepSeek and their new R1 model, and you know what does this mean for us. What does this mean for us as practitioners? What does this mean for us as industry? Should you be scared? What should you be scared of? Let's start off with you know what are you guys thinking?
Speaker 1:any initial thoughts on what you've seen, the model where you've seen other people talking about I can say that what this news came out within the last two days and it already feels like a week's worth of news for sure that's a good way to describe it.
Speaker 3:Honestly, I'm a little surprised of, like, what this is. What's busting the ai bubble a little bit. I'm I'm actually really surprised. This is what it is.
Speaker 3:But in my mind DeepSeek is more like hey, just don't turn your brain off and throw more NVIDIA chips at the problem. That's pretty much. They found a way to be more efficient in the training of the model that you don't need Sam Altman's trillions of dollars and a bunch of nuclear power plants to be able to run a bunch of larger models. Like, hey, hey, what about if we actually take a step back and try and be a little bit more efficient, versus just slamming our head against the wall? It's like that old analogy. You know, throw much eggs at the wall, one of them won't break. That's essentially. It's a rough analogy.
Speaker 3:But how, like the state of let's? How do we get more intelligent llm models? Let's just add more compute power and deep sea's? Like, well, because we actually can't use that many nvidia chips, because we can't import them into our country, let's find some more efficient ways to train language models. So it's more. Yes, it's not great for NVIDIA's current valuation, which was a little ridiculous anyway, but overall I don't remember exactly the rating, but it hit the top 10 of the global systems. Gemini is number one, so it's like it's not, and OpenAI beats it in things in Anthropic. So DeepSeek is not a revolutionary in being better than OpenAI or anybody else. They just did it cheaper. So I think that in the news cycle that's gotten lost a little bit, that it's not like some new general intelligent AI system.
Speaker 1:No, it's not as performant as what we have in the United States up until now, and I think it's important to realize that one of the things that has always been a thorn in the side of Gen AI and large language models that have been coming out from OpenAI and now DeepSeq is the context of which they're building them. There's all of these data points in models like OpenAI, but then DeepSeq really went into something specific to kind of build out. There's also regional context that came in. For example, if you put in a prompt about don't tell me a little bit about Tiananmen Square, it kindly tells you it doesn't really know anything about that from deep seek. So we just a reminder to everybody you have to be very, very aware of how these are being built and for what. Yeah, cool, it's cheap and it's using less compute and using less energy. But there's another cost. I think my chemistry professor put it to me very well In business and in chemistry there's no such thing as a free lunch.
Speaker 3:Well, in this case too, they are cheaper, but they're also sending all the information back to the Chinese government too. So, if you're not using the open source version, so yeah, it is cheaper, so it's going to start at a cost for me. Also, there's a cost to it being less expensive too, outside of it not telling you necessarily proper information.
Speaker 1:Yes, which fair is fair? Have that context going into some of the models built by OpenAI Anthropic? Like you, are at the mercy of what those are trying to do.
Speaker 3:Which is the bigger point, is they're not accurate anyway?
Speaker 2:So it's like to what extent does this like change the?
Speaker 3:game like who should be scared? It's open. Ai right, yes, they built their original moat.
Speaker 2:That's right. That competitive mode is gone now they built around this like super secret model. It's really expensive. You have to pay us to use it effectively. And that wall is down now. And does this pop the AI bubble?
Speaker 1:No.
Speaker 2:Does this pop OpenAI's strong, strong stranglehold of the competition? This is probably the first crack in that.
Speaker 3:And they are losing money now with, like, they're having to cut prices to keep up and they've never been making money, but now they're really losing money on just even hosting it. And also, NVIDIA is a little bit of a loser here too, because they've been kind of operating in analysts' kind of our limits, but they've been kind of following, like you, just the more AI is a thing, you're going to need more NVIDIA chips. Well, now, that's kind of like well, maybe you don't need unlimited NVIDIA chips. So for NVIDIA shareholders it's not the best, but they're not going anywhere. They're a great company and they have really useful products. It's just been overhyped that the only way to solve the problem is more chips.
Speaker 1:Right, yeah, and if we had been recording this yesterday it was like oh my gosh. Look at the market. Everybody put their money into NVIDIA. Wake up this morning. Oh no, it's okay.
Speaker 2:It's all back, everything's mostly fine. Yeah, I mean the NVIDIA cannot make enough chips to like. You know, nvidia should keep making chips, but let's not get crazy, for sure I remember these were graphic cards that were for video games is where they even came about Like they're still great for that.
Speaker 3:They are the world leader in those things and also other parts of AI and ML and things. People will use those. It's just the overhyped of nuclear power plants and unlimited NVIDIA chips. That's the only thing that's changed.
Speaker 2:Yeah.
Speaker 3:Yeah.
Speaker 2:Well, I think we're ready to hop into the episode then and this tees up really nicely because LLMs are what's being used to power these modern agent AIs, oh my.
Speaker 3:Oh boy.
Speaker 2:So I want to preface this with something kind of fun, right? So? There's a group called Artisan out there and if you live in New York City or San Francisco, you've seen these ads, and these ads are provocative. I'll read you four of my favorites here. Ais are excited to work 70 hours a week. Stop hiring humans. Hire Ava, the AI business development representative. Ais won't complain about work-life balance and hire AIs not humans.
Speaker 1:And can I just put one clarification on this Stop hiring H-I-R-R-I-N-G humans.
Speaker 2:Yeah, humans are the ones that make mistakes.
Speaker 1:For those of us that don't live in San Francisco or New York. We needed that clarification. But yeah, in a world that's constantly changing, we know technology is changing, but you've got things going on like this. They just like keep going surface level at people and like it's going to be this easy, it's going to be the silver bullet, it's going to be this easy. And now we're AI agents trying to do the exact same thing that LLMs did two years ago with the release of ChatGPT. Put it into context for us like agents and where this is going, yeah, so let's differentiate them quickly from older styles of modeling.
Speaker 2:So an old model you deploy it and you want it to predict some outcome. You want to predict the housing price tomorrow. You want to predict who is going to have an instance of diabetes. Maybe you want to create some text, maybe you want to write a poem for you or make an image for you, or maybe just aggregate a bunch of results for you. These are very, very clear. I have a problem. I want you to solve this specific problem, and it's a very narrow problem.
Speaker 2:We're asking these AI agents, which are built on the same LLM's mind these are not new models underneath the hood to do everything and everything a human would do, right. So you can think of this story as like if a human's given a problem, they perceive what's happening, they reason about the problem, they act on it and if anything new happened, they learn about what happened Right. So in the perceiving stage, the AI agent will gather all this data from multiple inputs or stimuli. Then it'll think about how do I solve this problem? In the case of an LL, an LM, you're gonna just ask the LLM or, like you know, your GPT. Think about this for a few seconds, tell me what you think you should do in the.
Speaker 2:In the past, when we had these types of models, like in econometrics models we would have a knowledge graph or a predictive machine learning model. That's kind of going out the room now. Now we have LLMs and especially when you think about you know people like dr Wolfe from, who's said things like LMs don't reason right. None of these models are built to reason. Even DeepSeek isn't meant to reason right. And then, with that reasoning, it'll then act in the real world via API calls or integrations and if you've developed some kind of feedback loop, it'll then learn from its mistakes or learn from things about its environment, right?
Speaker 3:So we're really asking these models we already have off the shelf to just act fully like an autonomous human employee which this, this use case describing, which I left as like um pid controller I'm not sure if you're familiar with that from control theory. So that's essentially if you think of, like um, your car's auto, not autopilot, but um, cruise control. Cruise control is essentially that it takes in an input it perceives like are you above or below what the set point is, and then it will adjust accordingly, like it's in basic thing I'm going to feedback loop, constantly going. So like basic things like that, that concept of also kalman filters and things we talked about before. These are old technologies that have been around and they have, when they're very simple, of what's the what is your goal? There? It's like, why are you adding, like that reason is very specific, like narrow ai agents is also, if you really boil it down, it's computer programs that's also not new the.
Speaker 3:The thing that's interesting here will be related to an ibm quote, a second. That's kind of solidifies. It's like why are we putting an llm in the middle of this? Because the idea of perceive, reason, act, learn that's standard. It's a great idea and I like that. We're coming back to that when we get into a little bit more of the roots later, of what actual agent-based modeling is, a concept that's been around for a long time. As I mentioned, in economics that is a very common methodology and there's different ways we could do this. The idea that we're building systems that are multi-step that is not new and it's a great thing. What's interesting is why are LLMs involved right now?
Speaker 2:I think the next quote that Sid will take us through helps us understand that a little bit more, so I'll try and summarize a little bit, just because it's a long quote. Agentic AI is basically being positioned as a way to save LLMs. Because LLMs are not making money for the organizations that are putting them out there, Agentic AIs give them a platform to say hey, you can hire an LOM to be one of your employees, and that would sure be a lot cheaper than hiring a real human employee. And so now here's the quote. For organizations and this is directly from IBM for organizations struggling to see the benefits of Gen AI, agents may be the key to finding tangible business value. Monolithic LMs are oppressive, but they are limited use case in the realm of enterprise AI. It has yet to be seen if all of the money being poured into LMs is going to be recouped as a real world use case. Agendic AI represents a promising framework that brings LMs into the real world.
Speaker 3:Wow, and IBM said that this is. What's funny is that Harvard Business Review has another article that's even more in depth of this. I'm like I want to timescale this thing. What is two years ago? When we first called that, we were kind of like calling foul on some of the LLM hype around two years ago when we started the podcast? I'd love the time layer. I need to look for it. Like, what did Harvard say about LLMs I bet they removed it from two years ago about how it was going to be this biggest thing for enterprises and use cases everything inverse of what IBM has now said of why you need to do agentic AI as the savior of gen AI. But gen AI was the thing that's supposed to be so helpful but it's not reasoning properly. So let's add an agent in there but still have it reason. So this is to me a little bit more like I would expect higher performance from just LLMs than trying to add multi-step. I think that's even confounding the number of errors you're going to be receiving.
Speaker 1:Yeah, and I think we're going to get into this a little bit later, like the deeper details of AI agents. But one of the things that strikes me about that, even from IBM's quote in this, is that I was talking with someone the other day like why, when does it just become apparent that AI agents aren't something that each individual will touch? It's just going to be the way software is built, if it hasn't already been happening already.
Speaker 3:Well, this is even basic programming, it's state-based machines. Like you'll have a state of a function, like even if you take it down to bits and bytes of binary coding things, you'll have an on and off. You'll know a lot of this, more than I will about how the parts work. But you just get to states. You have different states of the system and you put the states in a row and there's different decision points in each thing Markov chain. From my world I'm not a computer science background, Sid is but from economics you have to use Markov chains and state-based systems. So if you really boil that down, that's what software does. So this is not such a novel concept of having multi-state. And then we'll get into more later about balance equations and recursive dynamic programming, where you start at what's the end goal and you walk backwards. These are all concepts we've had for a long time.
Speaker 1:The only new thing here is deciding you need to put an LLM in there to save LLMs.
Speaker 2:Governance implications for AI agents yeah, so you might be tempted to say well, we got this AI agent, it's just like a normal employee, we don't have to govern employees, but you have to always govern your systems.
Speaker 2:So let's think about some of the expectations that you're going to see for AI agents to be properly used in governance settings where you care about it being correct, where it's touching your code bases. So in a recent state of AI agents released by LandChain, the number one issue that they cite is the performance qualities agents and how poorly these models do, as you expect them to do more and more and more steps. If you ask it a one-off question, that's fine. If you ask it something that takes four or five steps, these models quickly, quickly, quickly fall apart. It also has a lot of concerns about. You know. You have to make sure that you're checking the behavior of the system right. You have to make sure that does exactly what you asked it to do and that's not just acting in a way that's open and freely, because it's no longer a simple prediction. It can now make any change to your environment that it has access to.
Speaker 3:From a governance perspective, the fact that we have these multi-states and these more things, it becomes more complicated From even the basic like we are now getting as an industry our hands around, like if you do want to use LLMs and you basically want to accept that there's a 90% accuracy rate, even when you're using rags and things like that, the hallucination being an extra 10%. Or you ask it logic questions where it can't think, like how many elephants can fit in an Olympic-sized pool, questions like that and Gemini says it's barely one, that kind of thing. So the reasoning is the main gap they have and, as we've talked about before, it's because of how the systems are built, predicting the next word. We need to take a step back, not throw more NVIDIA chips at the problem and figure out a new paradigm to get more intelligent systems, but from a governance side, agentic AI compounds the governance considerations you have, because not only are you doing basic monitoring or fact-checking for performance, you now have to at every stage of the journey. The acceptable balance, because how agents are being touted is go book flights from me, go, do all these things and actually do human tests. So it's multi-step.
Speaker 3:So if you have a and if it misunderstands what you're asking it, then you already have an error there. So, hey, I want to go. Look to lisbon, portugal, what. And then it puts madrid, spain, in there. Okay, I'm already in the wrong country. And then, um, okay, maybe it gets the dates right, that you want to be there? Okay, cool, so they find that, but they sent out. And you want to marry your marriott members. They're going to find a marriott hotel. They're going to book you a Marriott hotel in the wrong country, but they didn't report back that it's okay. And then, like, if you don't catch, it's the wrong country, you now have a great deal, maybe even for Madrid when you're trying to go to Lisbon, and then the plane tickets who knows, you might go to Gibraltar, we don't know. So, like, you put all these things together and like, so you have to now have multi-step monitoring around the different states, as well as anomalous behavior, because what's also kind of concerning about these agentic ai is you're like we're going to give you right access for apis and things. So not only do you have first off the um, the additional segregation duties and things like that you have to have a talk but then anomalous behaviors of like you need to start installing credit limits and things like that because, for instance, if you got the wrong country when you're figuring out where to book your hotel well, currency is different conversion rates and maybe what a good deal is somewhere else is different than what your budget was and you have to start adding all of these intelligent constraints around where it's like you start.
Speaker 3:When you think about all the logic you have to do to put guardrails on, you could have just written a program in the first place. So, or a little bit, that's more of a part two of the podcast. We get into some like the utility-based theory and things like that of like we're from economics background, how agent-based models are normally used and they're used all over the world in federal, in banking systems and also for like for economic forecasting for policymakers and using logistics or using a lot of these different places like how do you have those, those step sequences? But now, with all these embedded controls and gatekeeping, then you need to have a human in the loop reviewing the results.
Speaker 3:There's so much additional downstream that when you see a great Salesforce ad at the Super Bowl about agentic AI, they're saying they have some famous actors and they're paying the money to say agentic AI is great. It was like my prediction is agentic AI will actually be used when it's not actually no AI is in there. Those build programs kind of like we know Facebook and other folks who have been using people to do the chatbot monitoring and things like that. So I think we'll actually I don't think agentic ai is going to go away. I think it's going to actually they're going to take the llm out and build deterministic programs, because when someone actually starts governing this stuff, realize it's more efficient to write a program than it is to figure out how to monitor the ai.
Speaker 1:And that's a stark contrast to what Gen AI was being pushed for in the first place, because this is something that could reduce your costs of resources to do anything like that. When, really, when you're looking for accuracy, you're looking for a lot more checks than with the speed of Gen AI.
Speaker 3:And that's what's surprising about the IBM quote. It's like why are they putting these things out there? You're thinking very short-term and not second order. Like you are inducing so many more issues. If you couldn't solve it with an LLM, you've now just created so much more of a headache for yourself. Internally compliance costs.
Speaker 2:Well, you know, we'll take a second here to pick on Cognition AI. They recently released Devon and it's a really cool idea for a project, right? Basically, this product is pitched as we built a virtual software engineer. You can hire the software engineer on a much cheaper license. The median salary right now for a software engineer is around $182,000, right.
Speaker 3:If Devon costs $10,000.
Speaker 2:Oh, that's not benefits or anything, either that's salary, that's just yeah, so if you can save 170, 170 000 by hiring devon, why wouldn't you? Right? And the way you talked to devon is like you just talk to them on slack like you would a normal engineer, right? That's the pitch here and you know I'm not trying to say that a cognition ai is specifically the problem. I think they're just an example of this like hole in the market being made by lms trying to apply agentic ai to them and then just seeing what happens. Uh, and you know, you know, their marketing material looks great and their benchmarks look great, I think the idea is great, by the way.
Speaker 3:Oh, the idea is great If this worked. Oh my gosh, yeah, wow.
Speaker 2:Yeah yeah. So you know it pitches this like tireless, skilled teammate, just like we heard about Artisan trying to claim it's made a bunch of fun web apps and extensions. But then it went into the hands of a group called Answer AI, and Answer AI is just like you know, any other AI organization and they said let's give it a go, we're in the business of trying this stuff out. And they did not like what they saw. To give a high level summary, they give it 20 different tasks, 20 standard software engineering tasks, and it failed 14 of them. It got inconclusive results on three of them and it succeeded on about three of them. So you know already not an incredible success rate. And when they then surveyed back their employees to say, like, how do you feel about using Devon? Was Devon easy to work with? You know they gave like the following quotes Tasks that seem straightforward often took days rather than hours at Devon, getting stuck in technical dead ends.
Speaker 2:It would produce overly complex and unusable solutions. It had a tendency to push forward with tasks that weren't possible, sometimes making up functionality for tools that did not exist. I mean, there's no way that we can't listen to this and just hear the same story that we've always heard for LLMs. The tool isn't any smarter than it is under the hood. The model is what the model is, and so everything you were concerned about before it's right here. But we gave it access to your APIs and to your repos. Now, no one said that Devon was catastrophic for them, but they found that they spent more time solving problems at Devon than if they had just done it themselves, which is not the outcome that we wanted here.
Speaker 3:It's wild. Yeah, it's like the multi-step compounding error problem where it used to be. You ask LLM a question. It will return a result. Maybe it's correct, maybe it's not. Maybe it'd help you with jam writing Like that's where I mean. The actual premise of LLMs can be helpful in some cases as a productivity tool. It's not what it's been told about but it's a helpful thing as a productivity tool.
Speaker 3:That's what we've been saying since day one. It's very helpful for specific things if a human's in the loop and you're using it just for productivity. But you are now taking what was petitionally helpful and making it completely unhelpful by adding multi-steps, because one little error compounds and compounds factorial combination and for coding even. We have gotten to this point where you have GitHub Copilot and these other tools. I'm doing informal surveys and I don't know how much you use it.
Speaker 3:I use it a little bit where I'm trying to find use cases for it. So, for instance, if I have a well-documented piece of code and I can ask it for doc strings, it does a pretty good job. Or if I have really well-defined functions, it can write unit tests for me that are relatively accurate but very trivial tasks and it can be helpful in that kind of thing. But asking it to build leave an example import a CSV file. One thing I've also noticed GitHub Copilot is not good with anything data science related. So if you ask it to do anything with pandas or any sort of data manipulation, horrible. So if it's not even working for that, who thinks you can have Devin actually be a software engineer? Did nobody actually test GitHub Copilot, and I've seen some crazy things about what github copilot is going to get rid of engineers and all this kind of stuff too.
Speaker 2:it's like, well, they're trying it, it's not working yeah, I mean, I think it is saving dev time. I think there are studies that like yeah, it does and like yeah, and it's like you know you're writing a rest api let it write the template for you and you fill it in.
Speaker 3:Oh, that's excellent but it's human in the loop. Productivity enhancer. It's very good for that, but also the thing that's weird is you actually have to be better to use it, because if you don't know what you're doing.
Speaker 3:You're gonna like this devon issue, you'll a small error the llm makes. That's inconsequential for sitter myself. If you don't know how to look at it and know that it's an issue, it snowballs into a big issue, which is what you're seeing with devon. Like little, it might mess up a little bit of logic early on and then it just snowballs Versus, like for Sid and I that know what we're trying to do, but it just saves us a little bit of time. Oh, catch quick error and go about your merry way and it saves you a lot of time. But that's where we're taking away the actual usefulness by adding it to the agent-based framework.
Speaker 1:Yeah, you and I had a conversation earlier today that we were talking about just the output of any of these, whether it's agents or just a regular LLM itself. You can start with it, but you have to be expert enough to finish it.
Speaker 1:You have to be able to finish it or make it unique or make it authentic, and that's, in some of the good ways, like creating a REST script or something to fill in, totally good, but we aren't going to see. That's not the applications that people are going. They're trying to go from zero to 100. And they wanted to do like, well, just finish this for me and be the finishing point, and it's like no, no, no, no.
Speaker 3:But that's why it's wild and that's where, like not to go too much tangent, but like our education system and everything like people aren't learning anymore and that's a bigger detriment of like. I'm concerned from like a software company perspective. In five years there's not gonna be any good junior developers. Let me make sure there's gonna be some, but like the quality of most people, because they're not gonna know they haven't done the hard yards and learned how to do anything. Same with like.
Speaker 3:I know your husband's a teacher, right, so like the, the quality of papers and something's. Everybody's having co-pilot write their papers and things like that. So it's like, but then no one's actually learning or being able to thinking because nobody really cares about what high school or college or whatever level papers. It's the thought process of you having to go through it and put your thoughts on paper is what the learning opportunity is, not that you actually delivered whatever grade, whatever paper, right, it's the thought process and the learning and we're like short changing people by just using the crutch yeah, quality of you said you brought to mind quality of thought and that's what we're missing with programming too.
Speaker 3:You're missing that ability to struggle with something and figure it out and, like you said, the zero to hero they're doing with like the agentic use cases. But let's actually just think about, like the flight booking, for an example, which I know. I don't know if that was a sales, but that wasn't a sales. Somebody I've heard like that as an example of it can be your personal assistant. You hire right For like using or business development representatives and things like that.
Speaker 1:Okay, well, let's take a step back.
Speaker 3:Like, some of those use cases are easier than booking a flight. But if you want to, by the way, tools like this already exist with like Expedia and things around. But like, okay, I want to go to this location. I want a virtual travel agent. Okay, so I want to go to XY location on these dates, cool, those the inputs.
Speaker 3:You have to put those inputs into an agentic ai anyway. So you're basically doing, say, a search result around all the areas to optimize for the lower cost is a linear programming optimization problem. That's been around since you know world war ii logistics of that's really when these these methodologies came about. Like, how do I optimize for a constraint, constraint being a cost and the dates you have? Those are two constraints you have to optimize for so you can look at all the potential hotel search results. You can put you to utility preferences. You might be a foodie, you might be someone that likes museums, like you can have some of your other things.
Speaker 3:So, within the city, try and go close to this. Like, how, even like a GPS or anything, works is take satellite inputs as well as people's user data that are in the app to see what's the most efficient way If you have a constraint. You don't want toll roads, that kind of thing too, and solving that. So you could easily make an agent quote, unquote, that is, a personal assistant for booking flights, and sell that and it has nothing to do with LLM and then it would absolutely crush it, because that's not actually a hard problem. Like I just walked through, by the way, I want credit or I want equity in whatever company.
Speaker 1:No, I'm just kidding, but you know what I mean.
Speaker 3:Like it's not that actual problem, but no one's really thinking about it that way.
Speaker 2:Yeah, yeah, I think that's totally fair. I mean, like you know, when we think about, like the agentic AI that works in our lives, that work well, think of Slackbot.
Speaker 3:Slackbot doesn't do a lot but it does it right.
Speaker 2:But Slackbot's great.
Speaker 3:This is the thing All of these tools are very helpful and you stack them together, you have productivity enhancements across the workforce. All of these things are good. I get the rap that I'm anti-LLM. I'm not anti-LLM. I'm anti the way a lot of people use them. I think they're very helpful for this specific thing. No-transcript make us more productive.
Speaker 2:Totally, and so I think, as we come to our conclusions and our final dots here, I think that I think we can pretty calmly say, like we said for the LMs agentic AIs based on LLMs are not ready for enterprise use as they require more and more tasks. They're just not up to creating the end results we need and creating things that we can actually maintain and take care of later.
Speaker 2:So, outside of a very few set of use cases, they're actually more painful and more annoying to use than traditional AI systems, because now you have to monitor every single step of what they do and you may be better served with as we're talking about a traditional agent-based or multi-step modeling paradigm.
Speaker 3:Yeah, and this will be an interesting one to watch and I'm less bullish with this. With even LLMs and we were like LLMs are by IBM and everybody else they're realizing that all these massive investments are paying off. And this is where it's just another tip of the iceberg thing with the deep seek and stuff is also showing like all the investments of all these companies, they don't have competitive moats and they've been wasting a lot of money. So like there is this weird. There is definitely an inflection point in AI right now, but I just think for the industry as a whole, agentic is the wrong direction and I hope somebody pivots soon because too much of a push sink the AI bubble right now in a bad way, versus like hey, we haven't figured it out, but let's retrench and figure out a new method. But going with like if we did energy-based systems or something else, if everybody puts all their chips in on a GenTIC, I'm concerned for the industry.
Speaker 1:Especially if they haven't even figured it out. If they broader they, if you haven't figured out how to govern or provide oversight over even simplest large language model, there's no way that you're ready for it.
Speaker 3:It's so much more complex and that's the thing I wish and we'll do a blog post and things like that around. This is the governance considerations. It really is an iceberg moment of you think, oh, even if it's useful some of the time, don't realize it that JITIC-ARI is so much more complicated to monitor and govern when companies, to your point, are still figuring out LLM governance. This is orders of magnitude more complex than LLM governance.
Speaker 1:This has been a great discussion. I know we were really excited to jump on this and maybe even a little calculated just to see how it played out over a couple days before we jumped on this topic. But once again, thank you for your thoughts today and for the listeners. If you have any questions for us on this topic or any of our previous episodes, please get in touch with us. We'd love to hear from you.